THE AI INDEX REPORT Measuring trends in Artificial Intelligence. STANFORD Institute for Human-Centered Artificial Intelligence (HAI)
The AI Index is an independent initiative at the Stanford Institute for Human-Centered Artificial Intelligence (HAI), led by the AI Index Steering Committee, an interdisciplinary group of experts from across academia and industry. The annual report tracks, collates, distills, and visualizesdata relating to artificial intelligence, enabling decision-makers to take meaningful action to advance AI responsibly and ethically with humans in mind.
The AI Index collaborates with many different organizations to track progress in artificial intelligence. These organizations include: the Center for Security and Emerging Technology at Georgetown University, LinkedIn, NetBase Quid, Lightcast, and McKinsey. The 2023 report also features more self-collected data and original analysis than ever before. This year’s report included new analysis on foundation models, including their geopolitics and training costs, the environmental impact of AI systems, K-12 AI education, and public opinion trends in AI. The AI Index also broadened its tracking of global AI legislation from 25 countries in 2022 to 127 in 2023.
Industry races ahead of academia.
Until 2014, most significant machine learning models were released by academia. Since then, industry has taken over. In 2022, there were 32 significant industry-produced machine learning models compared to just three produced by academia. Building state-of-the-art AI systems increasingly requires large amounts of data, compute, and money, resources that industry actors inherently possess in greater amounts compared to nonprofits and academia.
Performance saturation on traditional benchmarks.
AI continued to post state-of-the-art results, but year-over-year improvement on many benchmarks continues to be marginal. Moreover, the speed at which benchmark saturation is being reached is increasing. However, new, more comprehensive benchmarking suites such as BIG-bench and HELM are being released.
AI is both helping and harming the environment.
New research suggests that AI systems can have serious environmental impacts. According to Luccioni et al., 2022, BLOOM’s training run emitted 25 times more carbon than a single air traveler on a one-way trip from New York to San Francisco. Still, new reinforcement learning models like BCOOLER show that AI systems can be used to optimize energy usage.
The world’s best new scientist … AI?
AI models are starting to rapidly accelerate scientific progress and in 2022 were used to aid hydrogen fusion, improve the efficiency of matrix manipulation, and generate new antibodies.
The number of incidents concerning the misuse of AI is rapidly rising.
According to the AIAAIC database, which tracks incidents related to the ethical misuse of AI, the number of AI incidents and controversies has increased 26 times since 2012. Some notable incidents in 2022 included a deepfake video of Ukrainian President Volodymyr Zelenskyy surrendering and U.S. prisons using call-monitoring technology on their inmates. This growth is evidence of both greater use of AI technologies and awareness of misuse possibilities.
The demand for AI-related professional skills is increasing across virtually every American industrial sector.
Across every sector in the United States for which there is data (with the exception of agriculture, forestry, fishery and hunting), the number of AI-related job postings has increased on average from 1.7% in 2021 to 1.9% in 2022. Employers in the United States are increasingly looking for workers with AI-related skills.
For the first time in the last decade, year-over-year private investment in AI decreased.
Global AI private investment was $91.9 billion in 2022, which represented a 26.7% decrease since 2021. The total number of AI-related funding events as well as the number of newly funded AI companies likewise decreased. Still, during the last decade as a whole, AI investment has significantly increased. In 2022 the amount of private investment in AI was 18 times greater than it was in 2013.
While the proportion of companies adopting AI has plateaued, the companies that have adopted AI continue to pull ahead.
The proportion of companies adopting AI in 2022 has more than doubled since 2017, though it has plateaued in recent years between 50% and 60%, according to the results of McKinsey’s annual research survey. Organizations that have adopted AI report realizing meaningful cost decreases and revenue increases.
Policymaker interest in AI is on the rise.
An AI Index analysis of the legislative records of 127 countries shows that the number of bills containing “artificial intelligence” that were passed into law grew from just 1 in 2016 to 37 in 2022. An analysis of the parliamentary records on AI in 81 countries likewise shows that mentions of AI in global legislative proceedings have increased nearly 6.5 times since 2016.
Chinese citizens are among those who feel the most positively about AI products and services. Americans … not so much.
In a 2022 IPSOS survey, 78% of Chinese respondents (the highest proportion of surveyed countries) agreed with the statement that products and services using AI have more benefits than drawbacks. After Chinese respondents, those from Saudi Arabia (76%) and India (71%) felt the most positive about AI products. Only 35% of sampled Americans (among the lowest of surveyed countries) agreed that products and services using AI had more benefits than drawbacks.
Regional Comparison by Funding Amount
Once again, the United States led the world in terms of total AI private investment. In 2022, the $47.4 billion invested in the United States was roughly 3.5 times the amount invested in the next highest country, China ($13.4 billion), and 11 times the amount invested in the United Kingdom ($4.4 billion) (Figure 4.2.10).
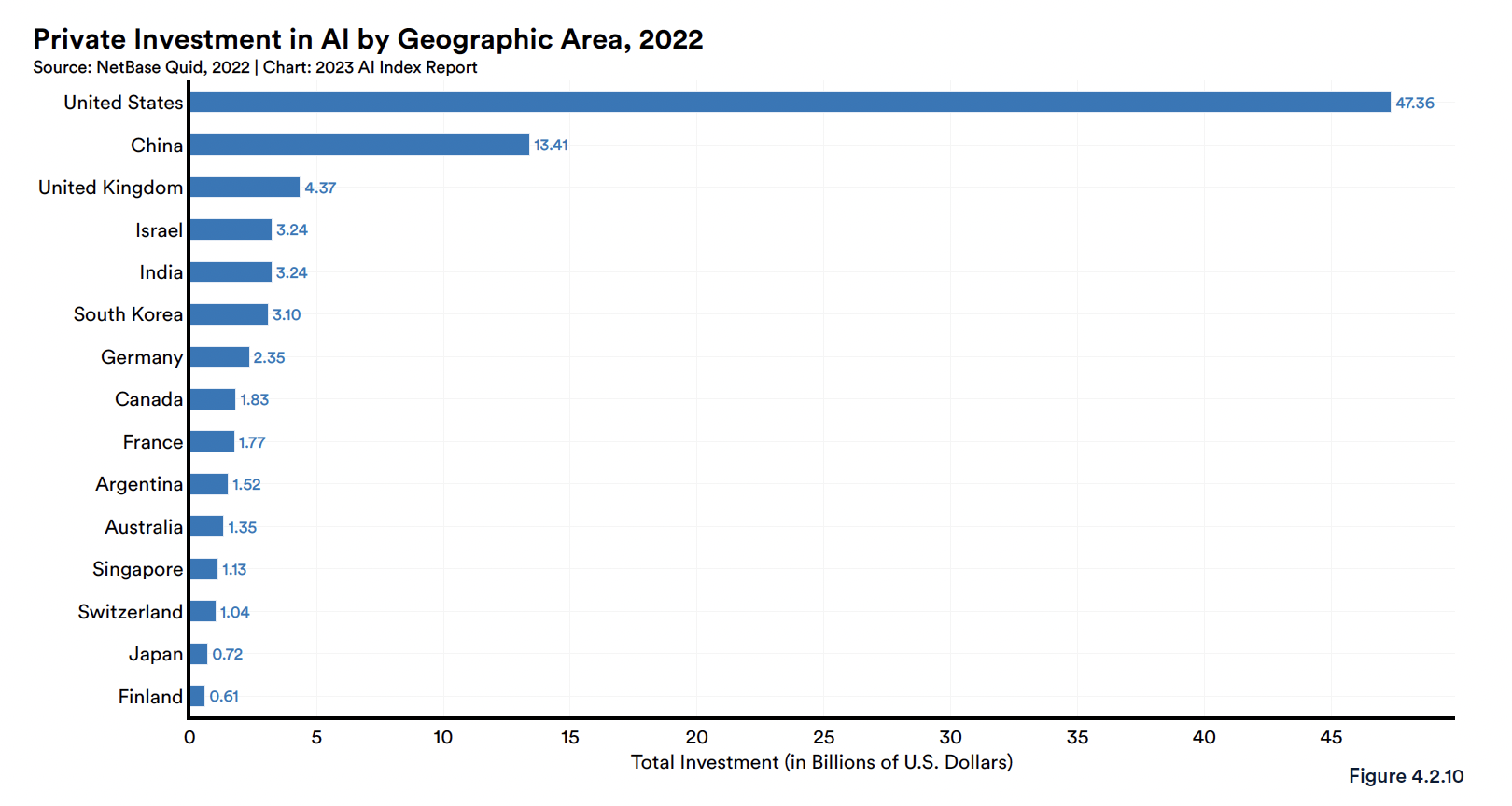
Supercomputer technology boom
The big AI Large Language Models (LLM) require supercomputer processing capability. Supercomputer technology power is on an exponential growth curve. The Graphics processing units (“GPU”) is a circuit commonly used for video games and enbles immense computing power for parallel processing of simultaneous tasks which is a key performance requirement for training AI LLMs.
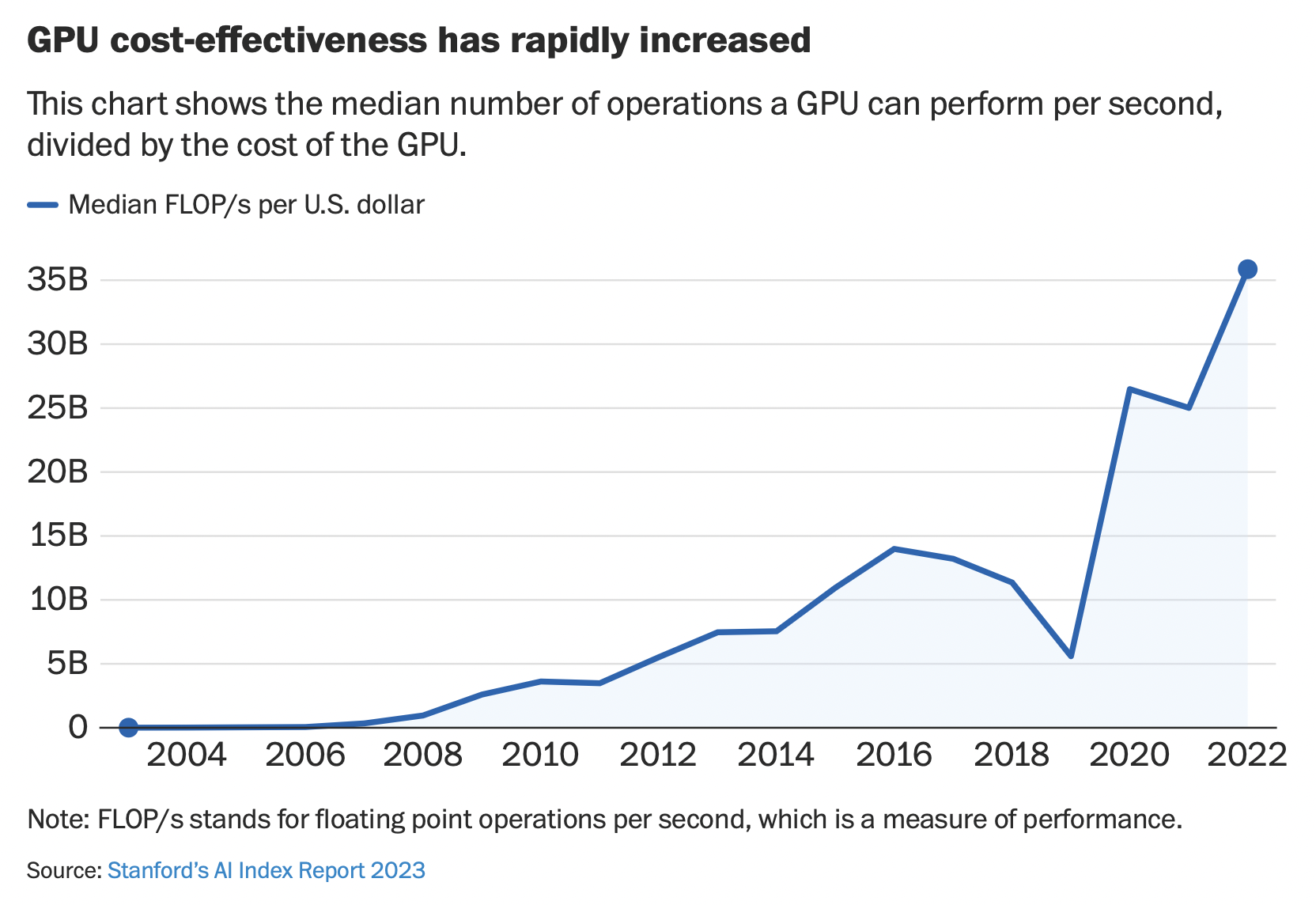
Learn More
Attention Is All You Need [Google 2017. the seminal neural network Transformer Model report]
- Abstract. The dominant sequence transduction models are based on complex recurrent or convolutional neural networks that include an encoder and a decoder. The best performing models also connect the encoder and decoder through an attention mechanism. We propose a new simple network architecture, the Transformer, based solely on attention mechanisms, dispensing with recurrence and convolutions entirely. Experiments on two machine translation tasks show these models to be superior in quality while being more parallelizable and requiring significantly less time to train. Our model achieves 28.4 BLEU on the WMT 2014 English- to-German translation task, improving over the existing best results, including ensembles, by over 2 BLEU. On the WMT 2014 English-to-French translation task, our model establishes a new single-model state-of-the-art BLEU score of 41.8 after training for 3.5 days on eight GPUs, a small fraction of the training costs of the best models from the literature. We show that the Transformer generalizes well to other tasks by applying it successfully to English constituency parsing both with large and limited training data.
See why AI like ChatGPT has gotten so good, so fast – The Washington Post (paywall)
- We asked three AI systems to generate content using the same prompt. The results illustrate how quickly the technology has advanced.