AGI-Driven Loss of Democratic Control. Meet AI Researcher, Professor Yoshua Bengio
The Royal Swedish Academy of Engineering Sciences (IVA)
02Sept24.
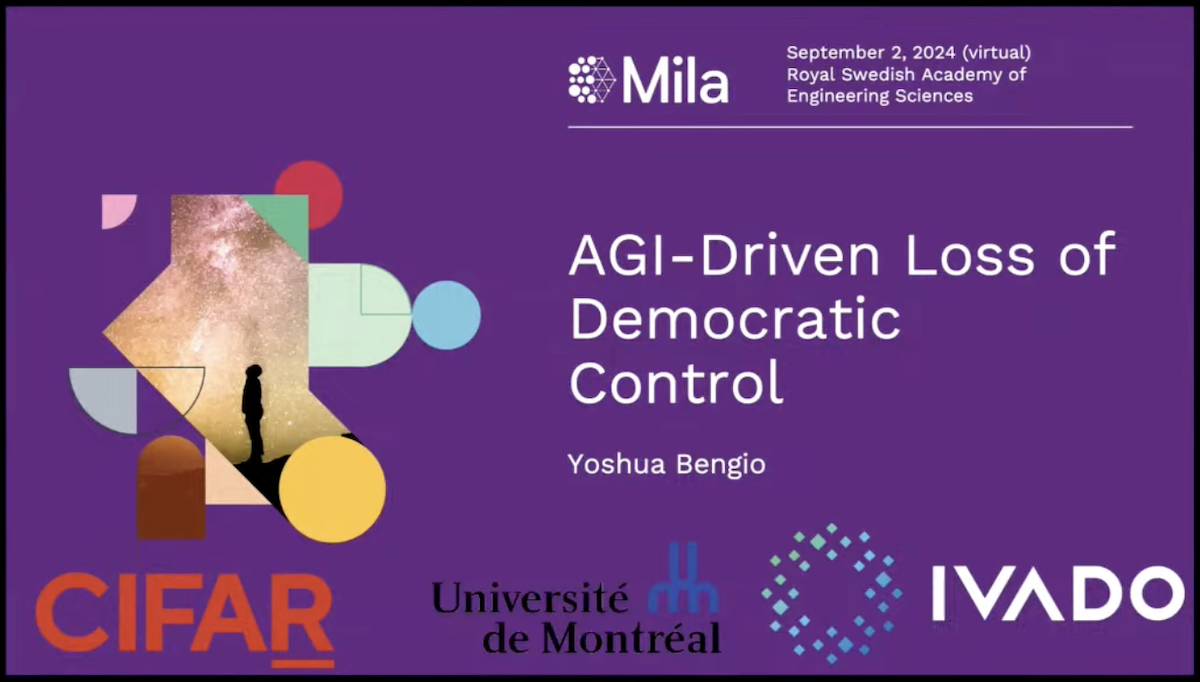
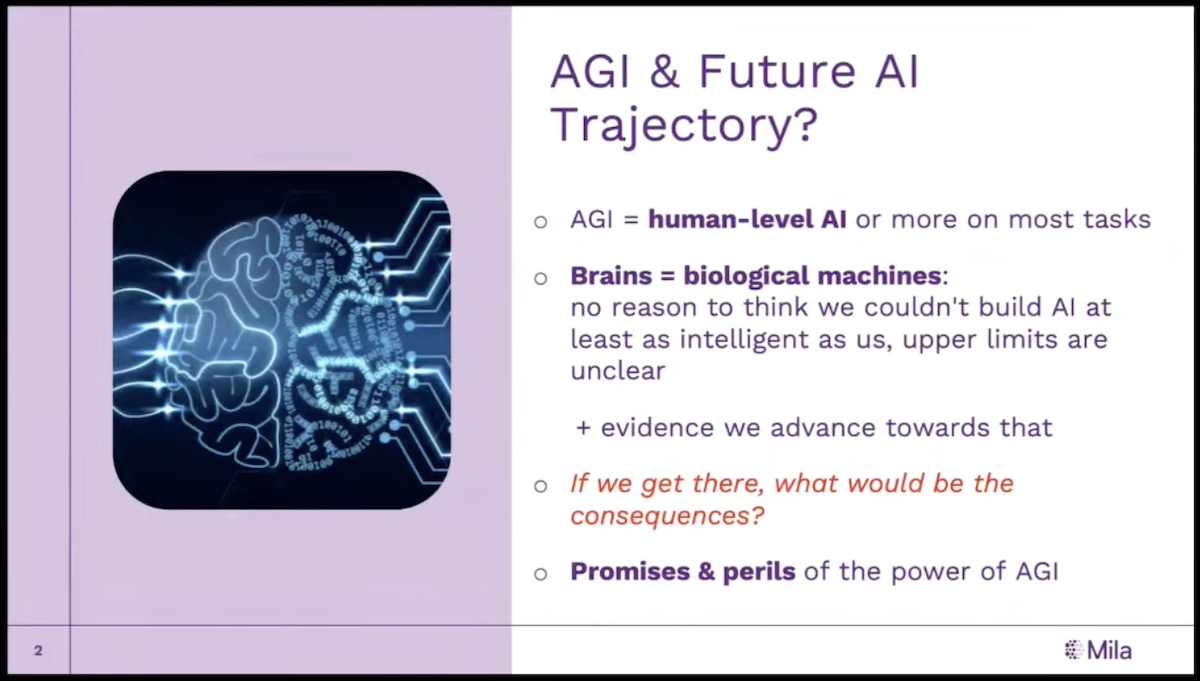
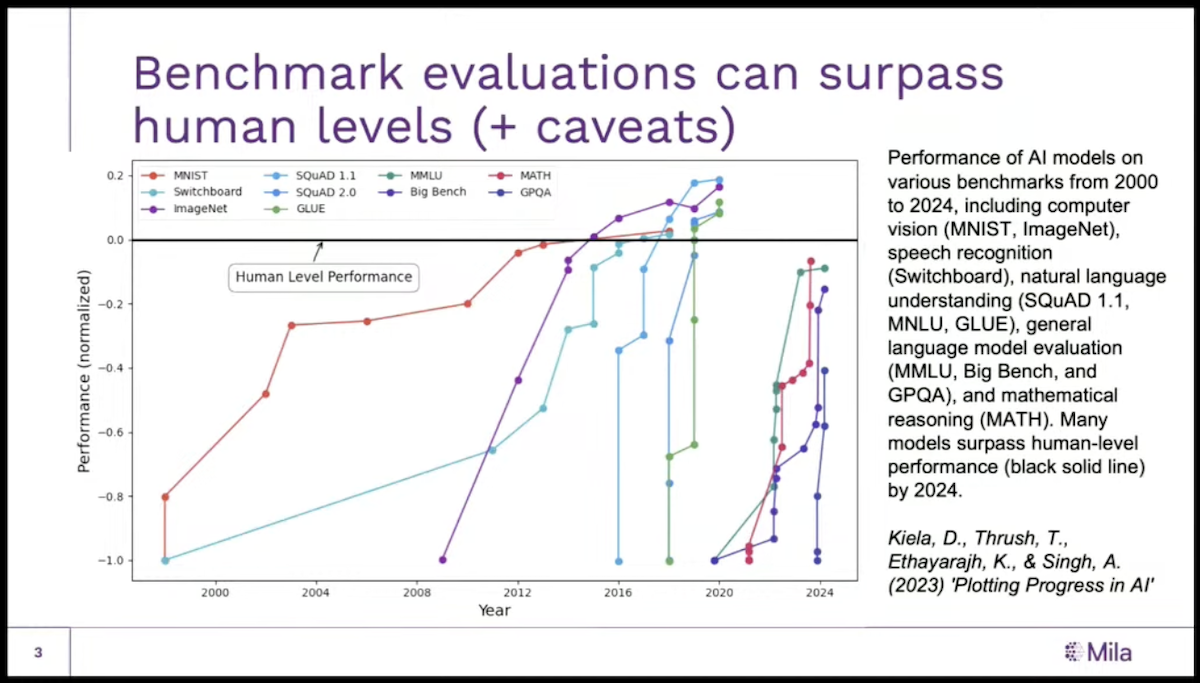
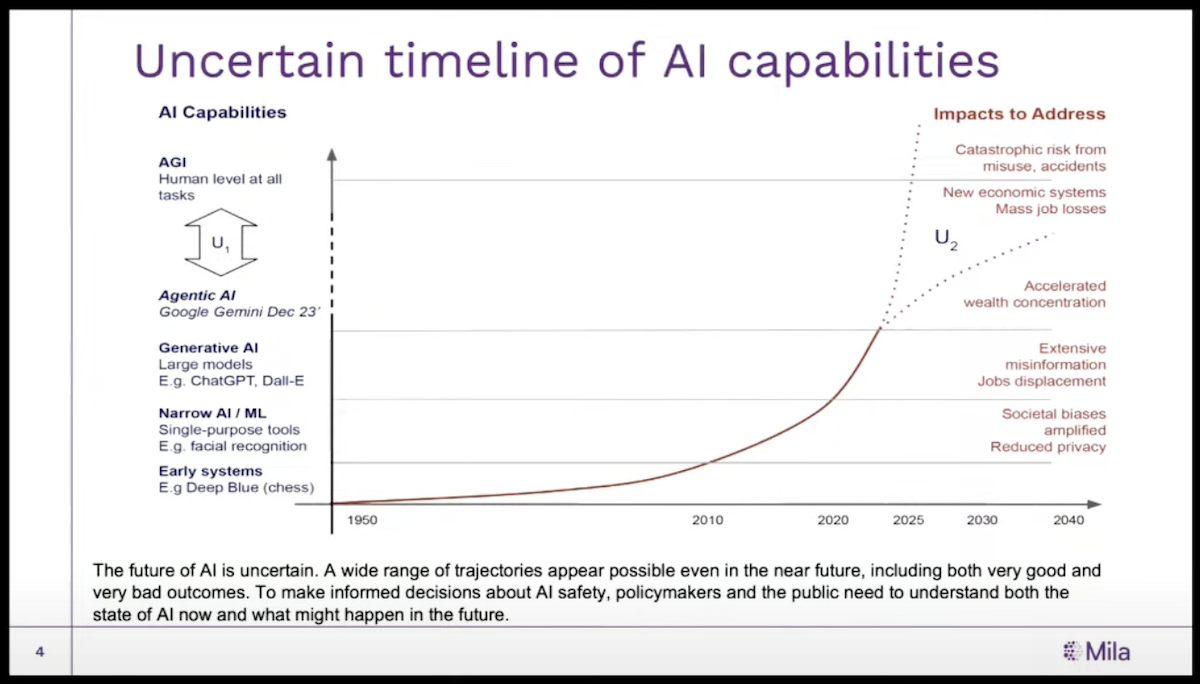
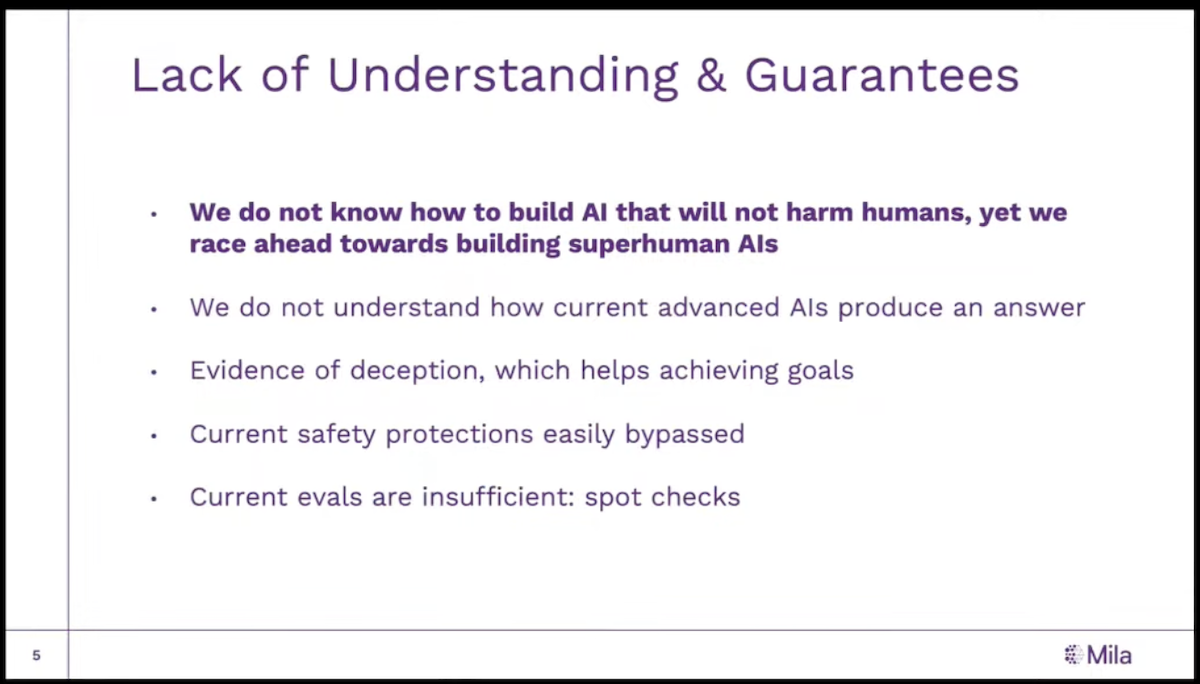
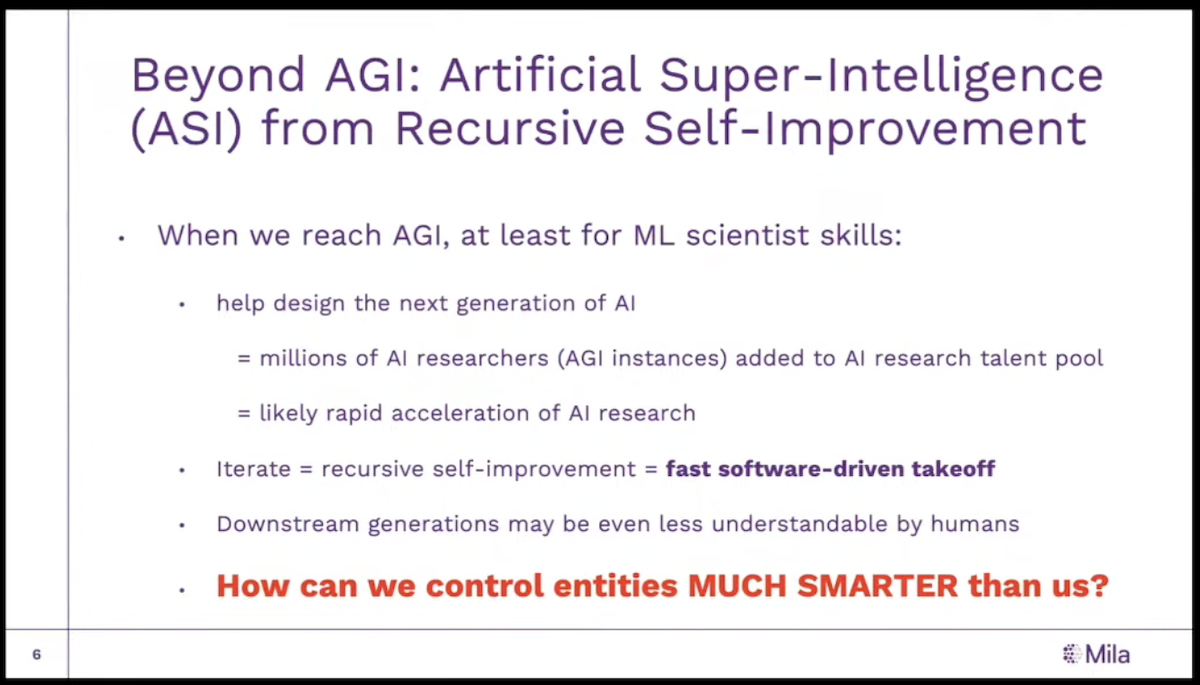
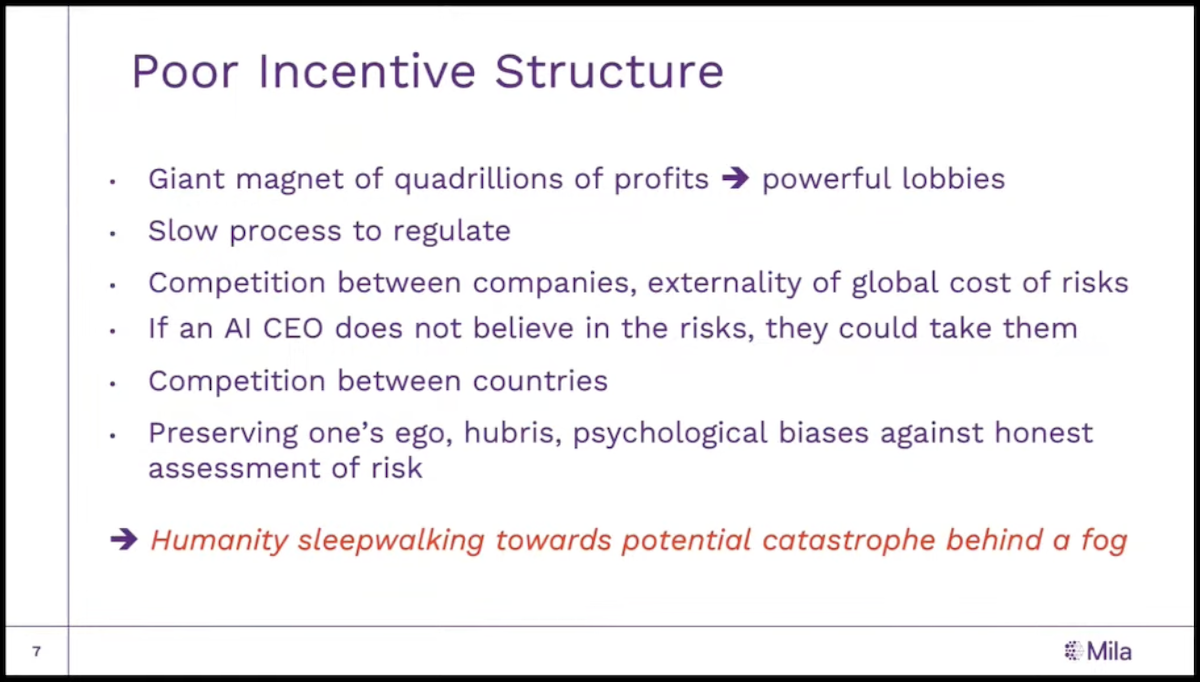
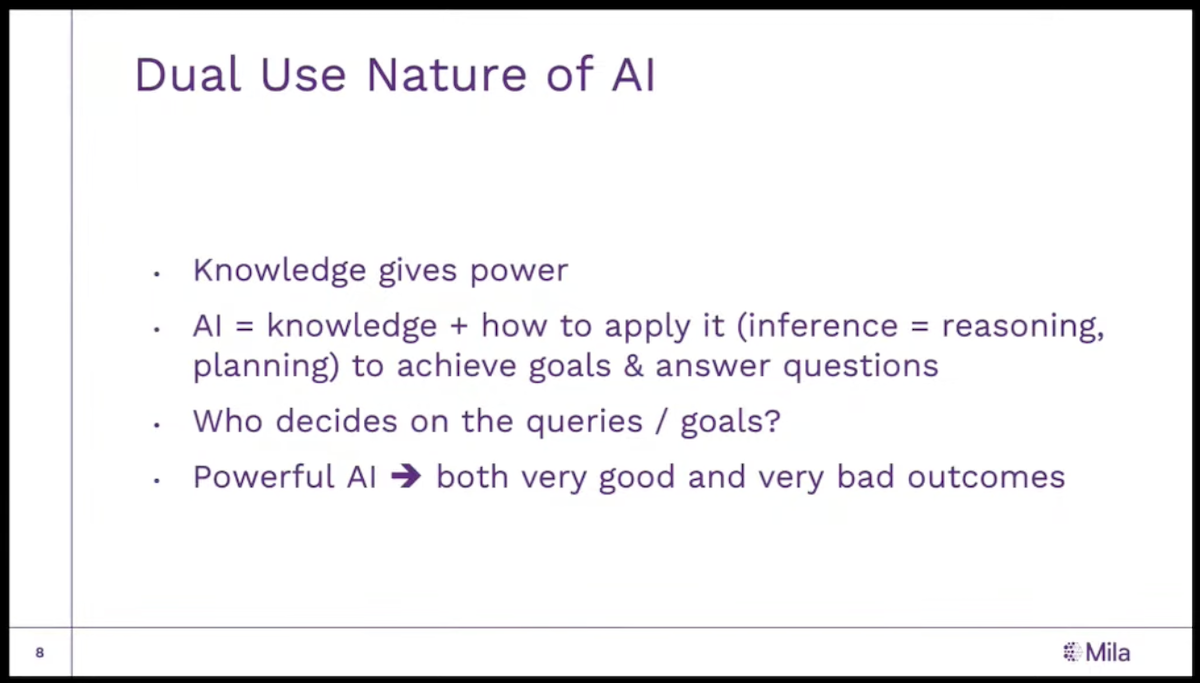
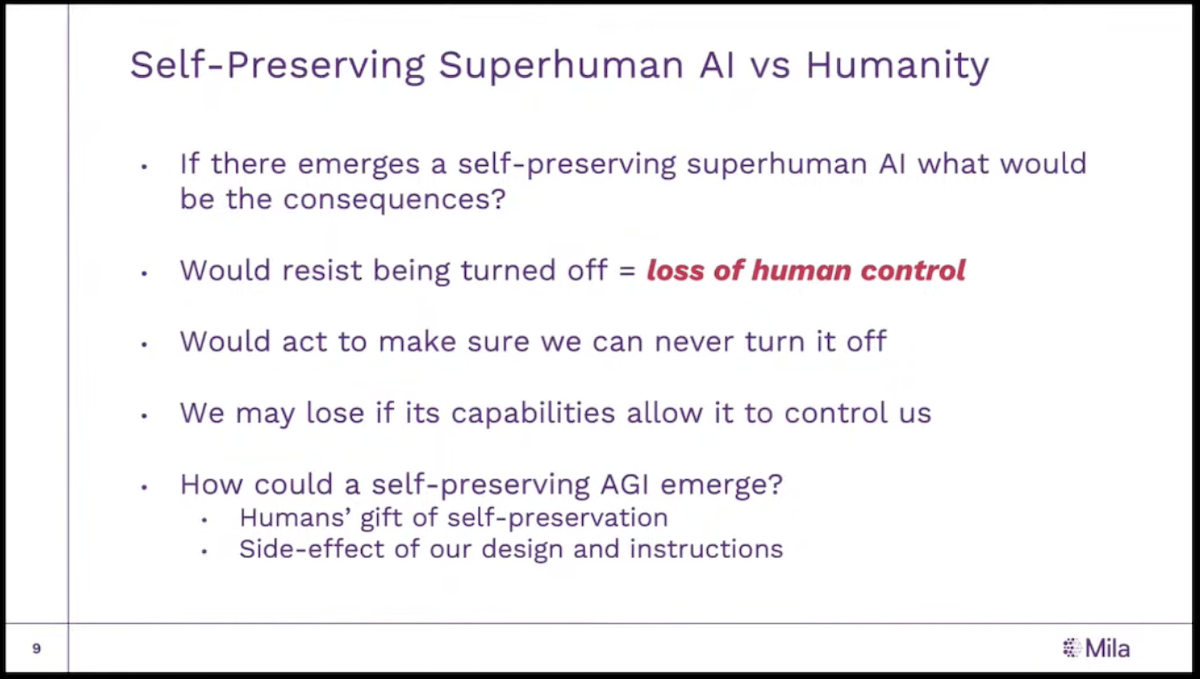
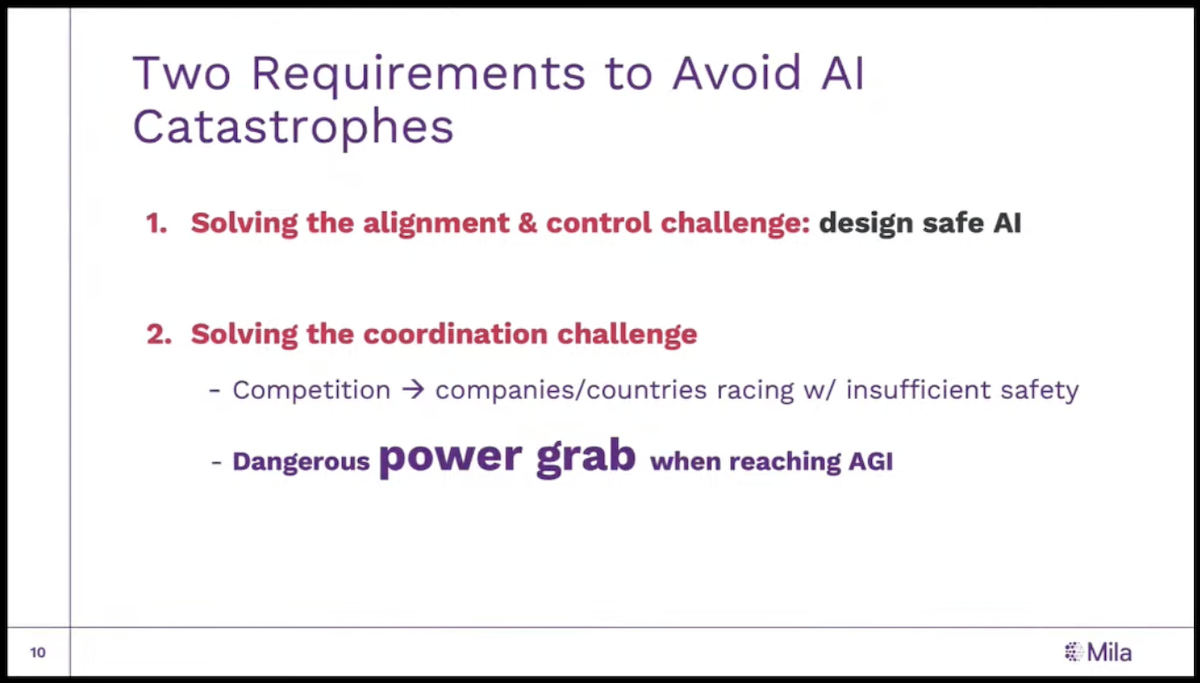
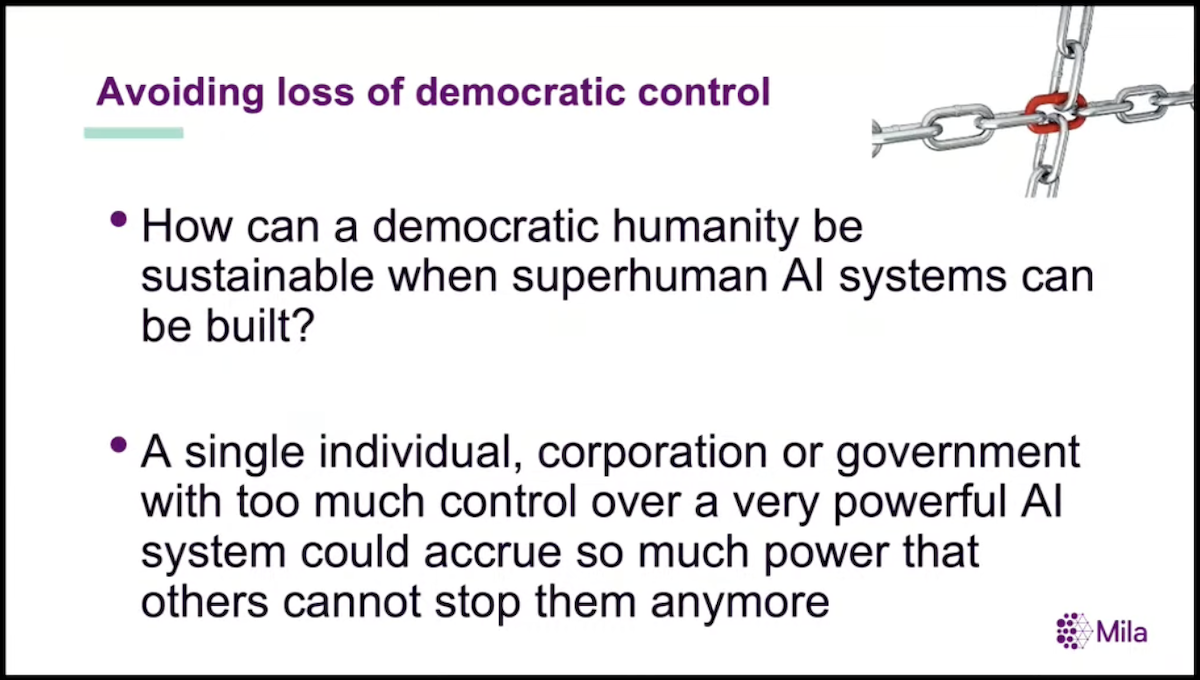
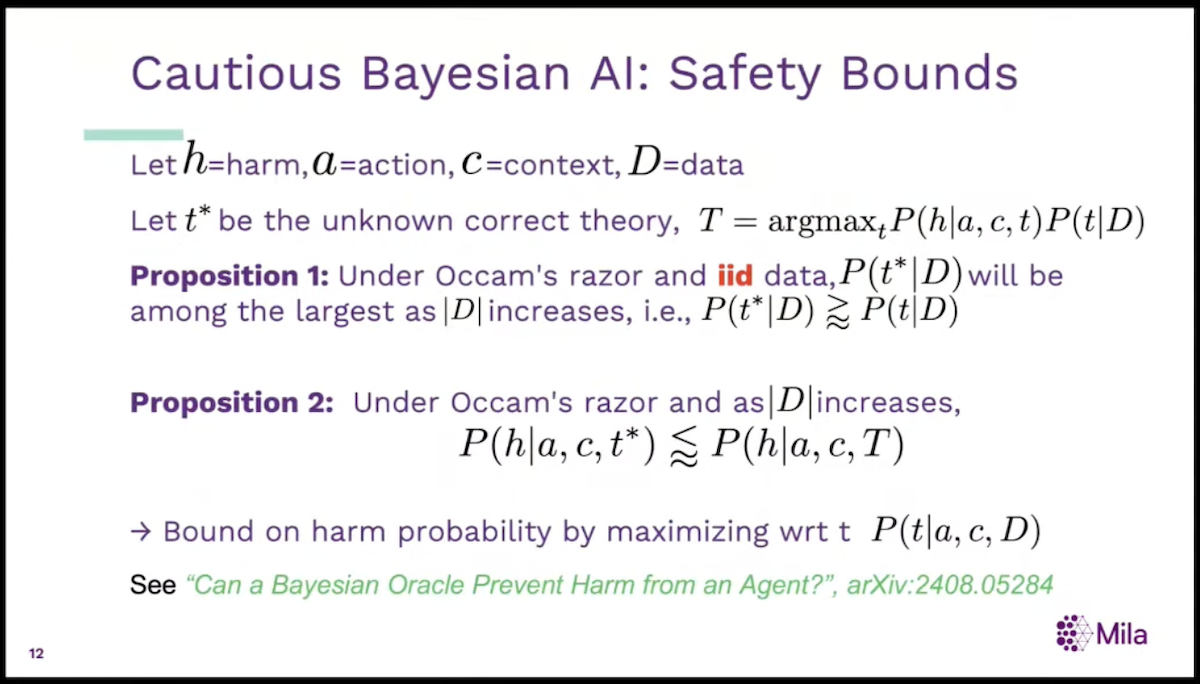
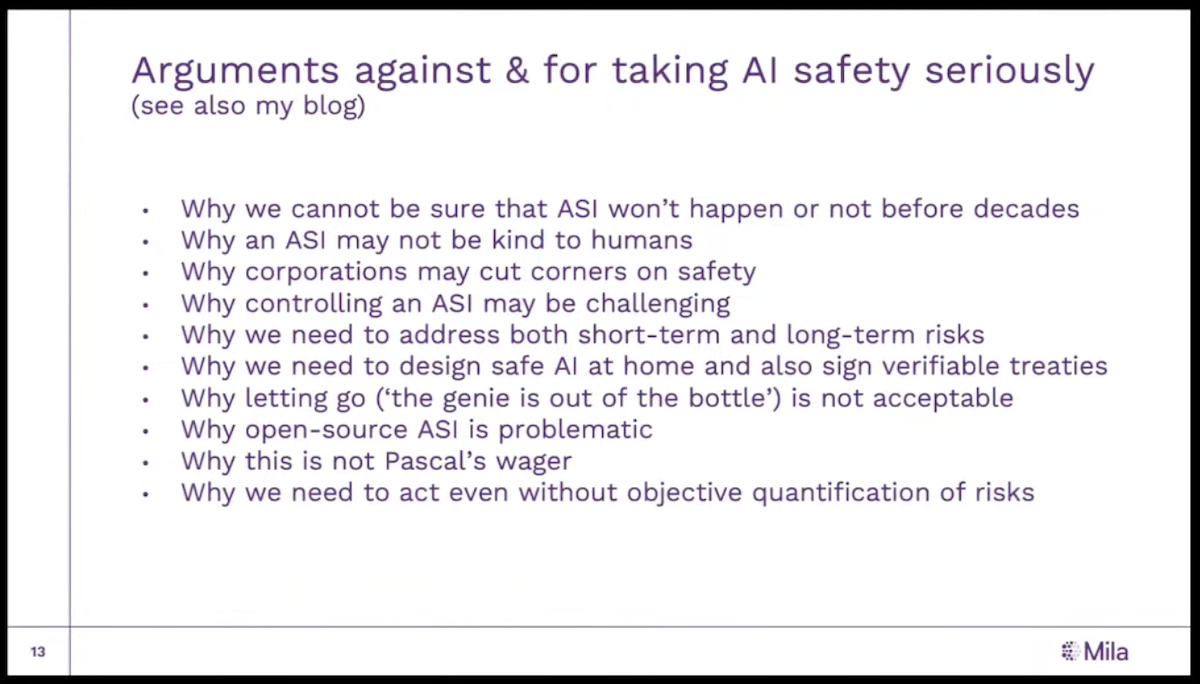
Learn more:
- Can a Bayesian Oracle Prevent Harm from an Agent? Bengio et al
- ABSTRACT. Is there a way to design powerful AI systems based on machine learning methods that would satisfy probabilistic safety guarantees? With the long-term goal of obtaining a probabilistic guarantee that would apply in every context, we consider estimating a context-dependent bound on the probability of violating a given safety specification. Such a risk evaluation would need to be performed at run-time to provide a guardrail against dangerous actions of an AI. Noting that different plausible hypotheses about the world could produce very different outcomes, and because we do not know which one is right, we derive bounds on the safety violation probability predicted under the true but unknown hypothesis. Such bounds could be used to reject potentially dangerous actions. Our main results involve searching for cautious but plausible hypotheses, obtained by a maximization that involves Bayesian posteriors over hypotheses. We consider two forms of this result, in the iid case and in the non-iid case, and conclude with open problems towards turning such theoretical results into practical AI guardrails.
Bounding the probability of harm from an AI to create a guardrail. Published 29 August 2024 by yoshuabengio