The new method of test time compute is a rapid strategy to compete with state-of-the-art models.
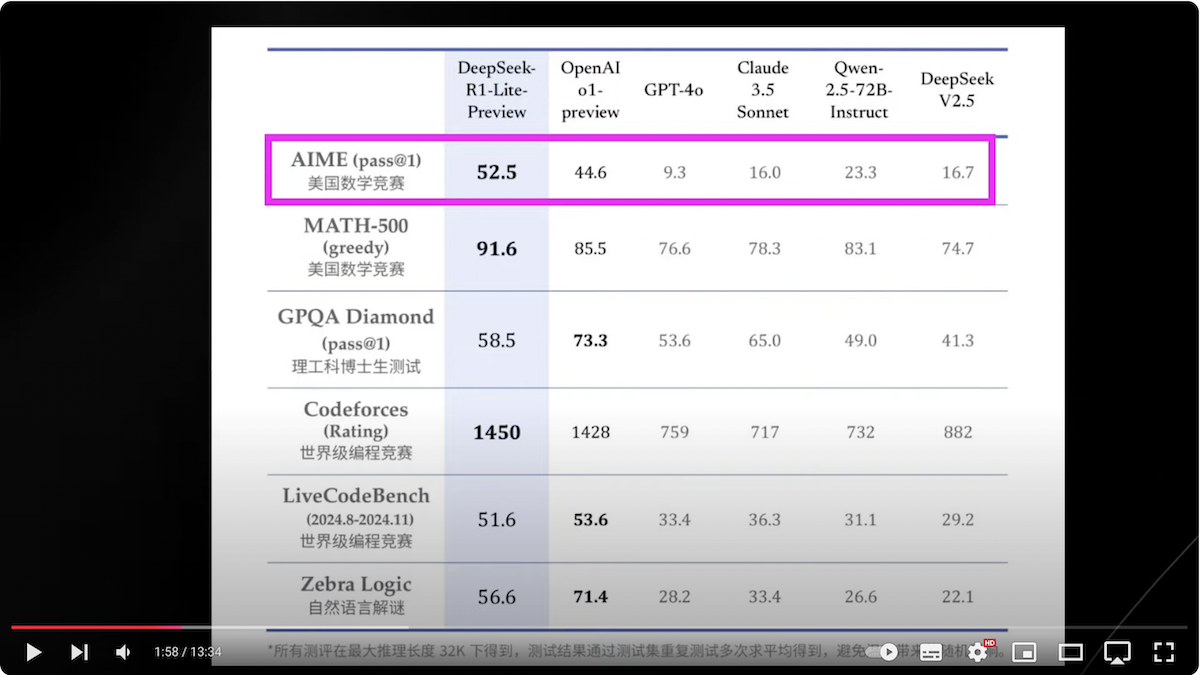
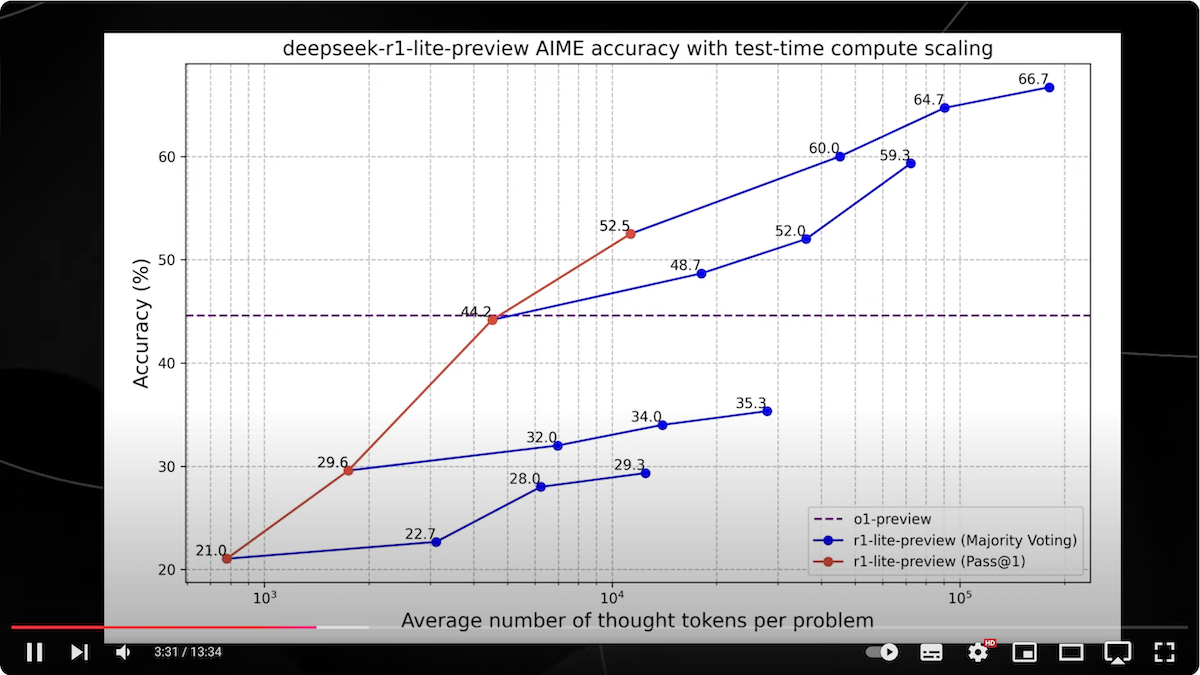
so incredibly open AI has got some serious competition coming out of China recently there was information coming out of this company called Deep seek Ai and they announced that their deep seek R1 light preview is now live and apparently has 01 preview level performance on some of the most challenging benchmarks this is something that literally took the industry by surprise because it was only 2 months ago that we got the release of 01 in its limited form such as 01 mini and of course 01 preview and those models demonstrated far Advanced reasoning capabilities far more than anything we have currently available from any other companies but of course China have decided to one up OPI by releasing their R1 light preview which of course you can use for free now this is going to be something that is absolutely incredible because many would have suspected that it would take at least a year or at least potentially eight months to catch up to what open ey has but apparently China has been able to do this within the span of 2 months so if you’re wondering who this company is there isn’t that much information about them publicly but I do know that deep seek AI was founded in 2023 and it’s a Chinese company that is focused on advancing AGI and the organization specializes in developing Cutting Edge AI models that excel in tasks such as coding mathematics reasoning and natural language processing and they’ve actually made some previous models including deep seek version 2.5 deep seek coda and those ones were actually achieving a top performance on major AI benchmarks now if we get into the recent benchmarks you can see that when we actually take a look at what we’re seeing here it’s truly incredible with as to how much progress they’ve made in such a short time we can literally see that the Deep seek all1 light preview manages to surpass 01 preview in several certain categories if we look at the aim Benchmark which is a math benchmark you can see that deep seek R1 light preview surpasses 01 preview at a 52.4 benchmark compared to 01 previews 44.6 we can also see on this Math 500 Benchmark it gets 91.6 And1 preview gets 85.5 on the GP QA it doesn’t match up to 01 previews but on code forces it’s a passes and on the other two open I 01 preview manages to excel now this is all incredible but I do think later on I’m going to talk about some Nuance to this because I don’t know how much compute they apply to each individual problem so I do think there’s potentially ways for them to get better results but it will be interesting to see how all of that works and if you wanted to see or screenshot something this is a screenshot of all of the current benchmarks like the most widely used ones and literally on the math benchmark you can see that deep seek R1 light preview gets state-of-the-art which is 91.6 surpassing 01 previews 85 .5 and I think it’s really incredible to see those bars if you see the dark blue and the light green one we can literally see that those two manag to surpass most of these other models in basically every category by a large jump which is really really surprising when we start to take a look at how this new method of test time compute is going to revolutionize AI so now this is probably the graph that everybody wants to see this is how these models scale with test time compute now if you’re unfamiliar with the test time compute Paradigm the long story short is that the more you allow a model to think or the more compute you allocate to certain problems the better these models get at being able to solve those specific problems which is why they call it test time compute and which is why right now there is this entirely new paradigm where you essentially have a different number of thought tokens but problem and that allows you to continually scaling and the craziest thing about this entire scaling law seems like it doesn’t seem to be any end to the results that you get from this so far the more thought tokens per problem that we add the better these models get at responding to extraordinarily difficult prompts now the reason I said this one is going to be particularly interesting is because if we actually take a look at this purple bar which is the 01 preview which is from open a we do see that the accuracy on the am Benchmark with test time compute scaling it stays consistent but of course right now I don’t think there’s a way for you to change the amount of thought tokens per a problem like you can’t say think about this problem hard so right now it’s just there but I will be intrigued to see how the accuracy does increase with opening eyes models so now when we actually look at this chart we actually break things down and we’re just going to just break it down for you in the simplest way possible the first thing that I do notice is of course the 01 preview level and that is of course the purple line the dash line going through the middle now this line basically shows where opening eye are on the Amy Benchmark I’m just going to call it the Amy Benchmark which is just a math benchmark and I think the only reason that the accuracy isn’t increasing here is because when you actually use these 01 models or these thinking models you don’t actually get to decide how many tokens it applies to each problem it just automatically applies a certain amount which basically means that for some questions it’s not going to think about it at all and for other questions it will decide to think about it quite a lot so this bench mark that you’re seeing on this graph right here you have to understand that it’s pretty different because we’re seeing an increase in number of tokens per problem for these other models so when we actually look at the R1 light we can see that this model is actually really good when it comes to this difficult Benchmark and what you can see in the blue because at first this confused me but then I just looked into it and it makes complete sense is that we have two methods of actually breaking down these benchmarks so you can see we’ve got two different ones blue and red the red is pass at one which is basically where you just ask the model question and it’s just pass at one try and then of course you get majority voting which is where you have I think around several I’m not entirely sure how many numbers you have but you have seever several responses so with the pass at one that’s just basically where we’re giving the AI one chance and then of course with the majority voting this is basically where you get multiple responses from the model and then based on those multiple responses you pick the one that frequently shows up as the winner so that’s why you can see that with majority voting and test time compute we get this extraordinary graph that just continues to go up with the amount of tokens per problem which shows us you like another incredible scaling law with this majority voting which is uh pretty incredible but all of this stuff is you know truly insane because it shows us that on the back end when we’re really trying to work through a lot of hard problems we’re actually going to have to burn through a stunning amount of tokens in order to get to the correct response but overall what we can see here is a pretty clear Trend the company deep seek AI have truly managed to catch up to where 01 preview is and consider ing the fact that they’re using things like majority voting on these benchmarks it does show us that there is no limit currently from what we can see I mean the graph continues to go up it seems like there’s no limit to how these scaling laws will end and some people if you been predicted that companies right now like deep seek are in the gpt2 era which means the next iteration of these models is quite likely to be completely incredible in terms of their performance because I’m wondering what if they managed to bake majority voting into the model so it intrinsically generates tons and tons of responses and then of course it picks the best one you could see that if that was something that was baked into the model then of course that would push the benchmarks even further so this is something that I think it’s absolutely gamechanging because this truly shows us that we have entered a new paradigm and the fact of the matter is is that open ey yes they’ve got a lead but it doesn’t seem like they’ve got one for long now there also is something that is truly fascinating that I wanted to talk about one of the things that open ey actually did which a lot of people had I guess you could say a disagreement with was the fact that they hid the chains of thought so essentially chains of thought are basically where if you’re thinking through a problem the user is essentially going to be able to see what the AI model is thinking about so for example if you say okay how would I make a cake from scratch things step by step um the model is going to be thinking okay what kind of cake do I need to ask the user what kind of cake let’s think about this uh maybe I should pick a common cake I’m going to pick a lemon cake all of those thoughts that the model is thinking up before it manages to respond OPI actually chose for their thinking model to completely hide that so if you use 01 preview or 01 mini you actually cannot see what the model is thinking you can see a very very short summary but you can’t actually see what the model is thinking now of course they say that this is something that they do not want users to be able to do they say we also do not want to make an unaligned Chain of Thought directly visible to users now I do think that they probably just did this for competitive advantages I mean they ideally don’t want people to see how their models are thinking in order to get really good responses on the benchmarks but the crazy thing about this and why I brought this up is the fact that deep seek they’ve actually shown the chains of thought and the chains of thought are absolutely incredible for these models and it kind of makes me think maybe just a little bit that they might be a little bit conscious but that’s for another video but let me show you guys what I’m talking about so I saw this on Reddit which is basically says deep seek R1 light is surprised by the third R in Strawberry so you can see here someone asked the very famous AI question which is how many RS there are in strawberry and you can see that the model thinks to itself saying okay this is not an R this is not an r Wait there’s a third R and you can see that the model is getting surprised by this letter in the word strawberry you can see right here at the top the model says wait a minute did I just count three Rs let me make sure I didn’t miss anything strawberry and you can see it literally says yeah that’s three hour in total but I feel like that’s too many for such short word maybe I’m miscounting or misreading the word which is a strange thing to say when you think about it I feel like this um when you’re talking about an AI model which um I’m not getting into the Consciousness debate trust me on that one but I still think that this internal Chain of Thought is just pretty incredible to read now we also got more comments from Reddit stating that this preview thinks for over 6 minutes and even GPT 40 and Claude 3 couldn’t solve their specific problem which is pretty remarkable because thinking for around 300 72 seconds is a whopping amount of tokens and a whopping amount of compute for something that is a very fascinating problem now I also got this example from Twitter I’ll leave a link to this one but you can see this one right here was pretty interesting it said write a grammatically correct sentence without using any letters more than once and it fought for 3 seconds and it was able to get a repeated sentence and it was able to get the correct sentence and then when you look right here you can see that 01 actually didn’t get this one right it said GLI jock quiz NS DX dwarf um and there were two o’s jock and two which is of course not great but so overall if you can actually come on to the website you’ll be able to see this entire internal reasoning and you’ll be able to just click this and you’ll be able to see exactly what the model is thinking and how it thinks through your prompt now now the reason I think this is one of the most powerful things is because this actually gives us the insight to how the model thinks and the reason this is just absolutely insane and I’ve recently done this is if we can actually identify where these models are going wrong with their thinking patterns on a certain problem we can actually craft prompts that are even better that allow them to solve the issue and thus get even better at prompt engineering so the user example that I’m showing here is a very popular prompt this one says assuming the laws of physics on earth a small marble is placed into a normal Cup and the cup is placed upside down on a table someone then takes the cup and places it inside a microwave where is the ball now explain your reasoning step by step so basically this is a question that tests to see if these models have a world model and tests to see if it understands that if you turn a cop upside down with a marble on it and you pick that cup up the marble essentially remains on the table but firstly when I actually spoke to this model it actually said that the marble is still inside the cup which is now placed inside the microwave and interestingly enough I thought hm maybe this is just about the prompt and I would argue that it is actually about the prompt and not about how smart the model is and the reason I was able to realize that is because when I looked through the chains of thought to during the prompt I could see that for some reason the model was thinking that the way how I was picking up the cup would actually carry the marble and all I did was I made some slight iterations to my prompt and you can see that it says that the marble is on the table outside the microwave so I think with this what the very best thing you guys can do with is that if your model is currently getting distracted or confused about certain things you can look exactly at what’s going on inside look at where it’s getting tripped up reorganize your prompt and then you can use that for other similar problems which I think has remarkable use cases so with that being said let me know if you guys are going to try out this model I think it’s absolutely crazy that deep seek have been able to catch up to open AI 01 that quickly and I do think that this is probably going to actually Force open AI to move even quicker because they definitely did not think that companies would be releasing these models that soon it was probably well known that the next models that were going to be coming were probably like clae 3 Opus and of course Google’s new model but now with this other Chinese company that are hot on the heels of op ey I think people are really really going to start accelerating in terms of the aggressiveness