397,394 views 23 Sept 2024 #CNBC
Elon Musk has big plans for how artificial intelligence can help to propel his businesses forward. The tech titan founded xAI, a new AI venture, in 2023 to develop large language models and AI products, like its chatbot Grok, as an alternative to AI tools being created by OpenAI, Microsoft and Google. In June, it was announced that xAI would build a multi-billion dollar supercomputer, called Colossus, in Memphis, Tennessee to carry out the task of training Grok. Musk thinks supercomputers can also transform Tesla from a car company to an AI and robotics company. In August, Musk teased Tesla’s AI supercomputer cluster called “Cortex” on X. Cortex is being built at Tesla’s Austin, Texas headquarters. Back in January, Tesla also announced that it planned to spend $500 million on a custom-built Dojo supercomputer in Buffalo, New York. The job of these supercomputers is to process and train AI models using the huge amounts of video and data captured by Tesla vehicles and improve Tesla’s suite of driver assistance features, which the company calls Autopilot, as well as its more robust Full Self-Driving or FSD system. Despite their names, neither Autopilot nor FSD make Tesla vehicles autonomous and require active driver supervision. But reaching full autonomy is critical for Tesla, whose sky-high valuation is largely dependent on bringing robotaxis to market, according to some analysts. Finally, supercomputers can also be used to train Tesla’s humanoid robot, Optimus. Watch the video to learn more about why Musk has been building a slew of supercomputers. Chapters 01:55 – Supercomputers and xAI 05:08 – Supercomputers and Tesla 11:03 – Challenges and future outlook
Tech titan Elon Musk is known for being a car guy, a rocket guy, a social media guy, and now he’s also a supercomputer guy. And Elon Musk says Tesla will spend well over $1 billion by the end of 2024 on building an in-house supercomputer known as Project Dojo. Although supercomputers look a lot like data centers, they’re designed to perform calculations and process data at extremely high speeds. Both of them are about scaling up to very large amounts of computation. However, like in a data center, you have a lot of small parallel tasks that are not necessarily connected to each other. Whereas for example, when you’re training a very large AI model, those are not entirely independent computations. So you do need tighter interconnection between those computations. And the passing of data back and forth needs to be potentially at a much higher bandwidth and a much lower latency. Musk wants to use the supercomputing power to improve Tesla’s autonomous driving capabilities, and finally deliver on the company’s years-long promise to bring robotaxis to market. Supercomputers are also needed to train Tesla’s humanoid robot Optimus, which the company plans to use in its factories starting next year. All in all, Musk says that Tesla plans to spend $10 billion this year on AI. Musk’s new AI venture, xAI, also needs powerful supercomputers to train xAI’s chatbot Grok, which directly competes with OpenAI’s ChatGPT and Google’s Gemini chatbots. Several of Musk’s supercomputer projects are already in development. In August, Elon Musk teased Tesla’s AI supercomputer cluster called Cortex on X. Cortex is being built at Tesla’s Austin, Texas headquarters. Back in January, Tesla also announced that it planned to spend $500 million to build its Dojo supercomputer in Buffalo, New York. Meanwhile, Musk just revealed that xAI’s Colossus supercomputer in Memphis, Tennessee was up and running. CNBC wanted to learn more about what Musk’s bet on supercomputers might mean for the future of his companies, and the challenges he faces in the ultra competitive world of AI development. You had supercomputers, if you go to any of the national labs, they’re used for everything from simulation materials to discovery to climate modeling, to modeling nuclear reactions and so on and so forth. However, what’s unique about AI supercomputers is that they are entirely optimized for AI. Musk launched xAI in 2023 to develop large language models and AI products like its chatbot Grok, as an alternative to AI tools being created by OpenAI, Microsoft and Google. Despite being one of its original founders, Elon Musk left OpenAI in 2018 and has since become one of the company’s harshest critics. In June, it was announced that xAI would build a supercomputer in Memphis, Tennessee to carry out the task of training Grok. It would represent the city’s largest multi-billion dollar capital investment by a new to market company in Memphis history. The announcement came at the heels of xAI securing $6 billion in series B funding, raising its valuation at the time from 18 to $24 billion. By early September, Musk announced that his training supercluster in Memphis, called Colossus, was online. The supercluster is powered by 100,000 Nvidia A100 graphics processing units, or GPUs, making it the most powerful AI training system in the world, according to Musk. He went on to say that the cluster would double in size in the next few months. These GPUs have been around for a while. They started off in laptops, in desktops to be able to offload graphics work from the core CPU. So this is an accelerator. If you go back sort of ten years or so, 15 years ago, online gaming was blowing up and people wanted to game at speed, and then they realized that having graphics and the general purpose of the game on the same processor just led to constraints. So it’s a very specific task to train a large language model and doing that on a classic CPU. You can. It works, but it’s one of those examples where the particular architecture of a GPU plays well for that type of workload. In fact, GPUs became so popular that chipmakers like Nvidia for a time had a hard time keeping up with demand. The fight for GPUs has even caused competition among Musk’s own companies. Musk’s social media company X and xAI are closely intertwined, with X hosting xAI’s Grok chatbot on its site, and xAI using some capacity in X data centers to train the large language models that power Grok. In December 2023, Elon Musk ordered Nvidia to ship 12,000 of these very coveted AI chips H100 GPUs from Nvidia to X instead of to Tesla when they had been reserved for Tesla. So he effectively delayed Tesla’s being able to continue building out data center and AI infrastructure by five six months. The incident was one example that shareholders used in a lawsuit against Musk and Tesla’s board of directors that accused them of breach of fiduciary duty. They argued that after founding xAI, Musk began diverting scarce talent and resources from Tesla to his new company. Musk defended his decision on X, saying that Tesla was not ready to utilize the chips and that they would have just sat in a warehouse had he not diverted them. Musk has gone as far as to suggest that Tesla should invest $5 billion into xAI. Still, Musk has big plans on how artificial intelligence can transform Tesla. In January, he wrote on X that Tesla should be viewed as an AI robotics company rather than a car company. Key to this transformation is Tesla’s custom-built supercomputer called Dojo, details of which the company first publicly announced during Tesla’s AI day presentation in 2021. There’s an insatiable demand for speed, as well as capacity for neural network training. And Elon prefetched this in a few years back, he asked us to design a super fast training computer, and that’s how we started Project Dojo. During the company’s Q2 earnings call last year, Musk told investors that Tesla would spend over $1 billion on Dojo by the end of 2024. A few months later, Morgan Stanley predicted that Dojo could boost Tesla’s value by $500 billion. Dojo’s main job is to process and train AI models using the huge amounts of video and data captured by Tesla vehicles. The goal is to improve Tesla’s suite of driver assistance features, which the company calls Autopilot, as well as its more robust Full Self-Driving, or FSD system. They’ve sold what is it, 5 million plus cars? Each one of those cars typically has eight cameras plus in it. They’re streaming all of that video back to Tesla. So what can they do with that training set? Obviously they can develop full self-driving and they’re getting close to that. Despite their names, neither Autopilot nor FSD make Tesla vehicles autonomous and require active driver supervision, as Tesla states on its website. The company has garnered scrutiny from regulators who say that Tesla falsely advertised the capabilities of its autopilot and FSD systems. A 2024 report by the National Highway Traffic Safety Administration also found that out of the 956 Tesla crashes the agency reviewed, 467 of those could be linked to Autopilot. But reaching full autonomy is critical for Tesla, whose sky-high valuation is largely dependent on bringing robotaxis to market, analysts say. The company reported lackluster results in its latest earnings report and has fallen behind other automakers working on autonomous vehicle technology. These include Alphabet-owned Waymo, which is already operating fully autonomous taxis commercially in several U.S. cities, GM’s Cruise and Amazon’s Zoox. In China, competitors include Didi and Baidu. Tesla hopes Dojo will change that. According to Musk, Dojo has been running tasks for Tesla since 2023, and since Dojo has a very specific task: to train Tesla’s self-driving systems, the company decided that it’s best to design its own chip, called the D1. This chip is manufactured in seven nanometer technology. It packs 50 billion transistors in a miserly six 45 millimeter square. One thing you’ll notice 100% of the area out here is going towards machine learning, training and bandwidth. This is a pure machine learning machine. For high-performance computing, it is very common to have supercomputers that have CPUs and GPUs, However, increasingly, AI supercomputers also contain specialized chips that are specially designed for AI workloads, and the Dojo D1 is an example of that. One of the key things that came through when I was looking at D1 is latency. It’s training on a video feed that’s coming from cameras in cars. So the big thing is kind of, how do you move those big files around and how do you handle for latency? Aside from the D1, which is being manufactured by Taiwanese chipmaker TSMC, Tesla is also designing the entire infrastructure of its supercomputer from the ground up. Designing a custom supercomputer gives them the opportunity to optimize the entire stack, right? Go from the algorithms and the hardware. Make sure that they are designed to work perfectly in concert with each other. It’s not just Tesla, but if you see a lot of the hyperscalers, the Googles of the world, the Metas, the Microsofts, the Amazons, they all have their own custom chips and systems designed for AI. In the case of Dojo, the design looks something like this. 25 D1 chips make up what Tesla calls a training tile. With each tile containing its own hardware for cooling, data transfer and power, and acting as a self-sufficient computer. Six tiles make up a tray and two trays make up a cabinet. Finally, ten cabinets make up a hexapod, which Tesla says is capable of 1.1 exaflops of compute. To put that into context, one exaflop is equal to 1 quintillion calculations per second. This means that if each person on the planet completed one calculation per second, it would still take over four years to do what an exascale computer can do in one second. It is impressive, right? But there are other supercomputers, certainly, that are performing in that ballpark as well. One of those supercomputers is located at the Department of Energy’s Oak Ridge National Laboratory in Tennessee. The system, called Frontier, operates at 1.2 exaflops and has a theoretical peak performance of 2 exaflops. The supercomputer is being used to simulate proteins to help develop new drugs, model turbulence to improve the engine designs of airplanes and create large language models. The next generation of zettascale supercomputers are already in development. A zettaflop supercomputer has a computing capability equal to 1000 exaflops. As for Dojo, Dickens says its utility could go beyond turning Teslas into autonomous vehicles. If you wanted to train a robot on how to dig a hole, how many Tesla cars have driven past somebody digging a hole on the side of the road? And could you then point that at Optimus and say, hey, I’ve got hundreds of hours of how people dig holes? I want to train you as a robot. I know how to dig holes. So I think you’ve got to think wider of Tesla than just a car company. At a shareholder meeting this summer, Musk claimed that Optimus could turn Tesla into a $25 trillion company. But not everyone is convinced. It’s a daydream of robots to replace people. It’s a lofty goal. The price points, you know, don’t sound too logical to me, but, you know, it’s a great aspirational goal. It’s something that could be transformational for humanity if we make it work. EVs have worked. I just call me a very, very heavy skeptic on this robot. Despite all this potential for Musk’s supercomputers, the tech titan and his companies have quite a few challenges to overcome as they figure out how to scale the technology and use it to bolster their businesses. One such challenge is securing enough hardware. Although Tesla is designing its own chips, Musk is still highly dependent on Nvidia’s GPUs. For example, in June, Musk said that Tesla would spend between $3 and $4 billion this year on Nvidia hardware. Here he is talking about the supply of Nvidia chips during Tesla’s latest financial results call. We are seeing is that demand for Nvidia hardware is so high that it’s often difficult to get the GPUs. I guess I’m quite concerned about actually being able to get state-of-the art Nvidia GPUs when we want them, and I think this therefore requires that we put a lot more effort on Dojo in order to ensure that we’ve got the training capability that we need. Even if Musk did have all the chips he wanted, not everyone agrees that Tesla is close to full autonomy, and that Dojo is the solution to achieving this feat. Unlike many other automakers working on autonomous vehicles, Tesla has chosen to forgo the use of expensive lidar systems in its cars, instead opting for a vision only system using cameras. The issues in FSD are related to the sensors on the cars. People who drive these vehicles report phantom obstacles on the road, where the car will just suddenly brake, or the steering wheel will tweak you aside where you’re dodging something that doesn’t exist. If you imagine a white tractor trailer truck that falls over and it’s a cloudy day, you’ve got white on white and a scenario that is not very easily computed and very easily recognized. Now, a driver that’s paying attention is going to see this and hit the brakes hard. You know, a computer, it can easily be fooled by that kind of situation. So for them to get rid of that, they need to add other sensors onto the vehicles. They’ve been vehemently against lidar. They need to change the fundamental design. Dojo is not going to fix that. Dojo is just not going to fix the core problems in FSD. At times, even Musk has questioned Dojo’s future. We’re pursuing the dual path of Nvidia and Dojo. Think of Dojo as a long shot. It’s a long shot worth taking because the payoff is potentially very high. But it’s not something that is a high probability. It’s not like a sure thing at all. It’s a high risk, high payoff program. And then there are the environmental concerns. Supercomputers like those being built by Musk and other tech giants, require massive amounts of electricity to power them, even more than the energy used in conventional computing and an exorbitant amount of water to cool them. For example, one analysis found that after globally consuming an estimated 460 terawatt hours in 2022 datacenters’ total electricity consumption could reach more than 1000 terawatt hours in 2026. This demand is roughly equivalent to the electricity consumption of Japan. In a study published last year, experts also predicted that global AI demand for water may account for up to 6.6 billion cubic meters of water withdrawal by 2027. This summer, environmental and health groups said xAI is adding to smog problems in Memphis, Tennessee, after the company started running at least 18 natural gas burning turbines to power its supercomputer facility without securing the proper permits. xAI did not respond to a CNBC request for comment. But beyond supply chain issues and environmental concerns, some question whether supercomputers and AI are good business. Tesla is a company with car manufacturer problems with AI and robotics aspirations. They’re not about to make any money from AI anytime soon, and this FSD that was promised five years ago isn’t really about to happen either. Dojo is a massive project. It’s interesting and exciting, and it could open tremendous frontiers for them. It’s just I think we need to be skeptical, right? How does someone make money with this? There’s no visibility on that whatsoever. It seems like, you know, a shot in the dark. Instead, Irwin suggests that Musk stick to what he knows: making EVs. I’m very bearish on the stock. I see the fundamental value in EVs, right. If Tesla goes into Thailand and India and they invest billions in India, the supply chain will bounce into existence. They’re going to be a cost leader in the world. You know, they need to get out there with a mini car. So if they do, the outlook for the company will change. But Dickens is more positive. I think Tesla is changing the supercomputer paradigm here because the scale of their investment, the deep pockets that they’ve got and their ability to put all that investment to a single use case. Now, you can argue that FSD has been promised for a long time, and you can think what you want to think of Elon, all of the above is valid, but they are ahead, and they’ve already built a moat that the likes of GM, Stellantis and Ford won’t get close to any time soon or ever.
“In the case of Dojo, the design looks something like this. 25 D1 chips make up what Tesla calls a training tile. With each tile containing its own hardware for cooling, data transfer and power, and acting as a self-sufficient computer. Six tiles make up a tray and two trays make up a cabinet. Finally, ten cabinets make up a hexapod, which Tesla says is capable of 1.1 exaflops of compute.” [25 D2 chips/tray x 12 trays/cabinet x 10 cabinets/rack = 3000 D2 chips per “hexapod” rack]
D2 Chip
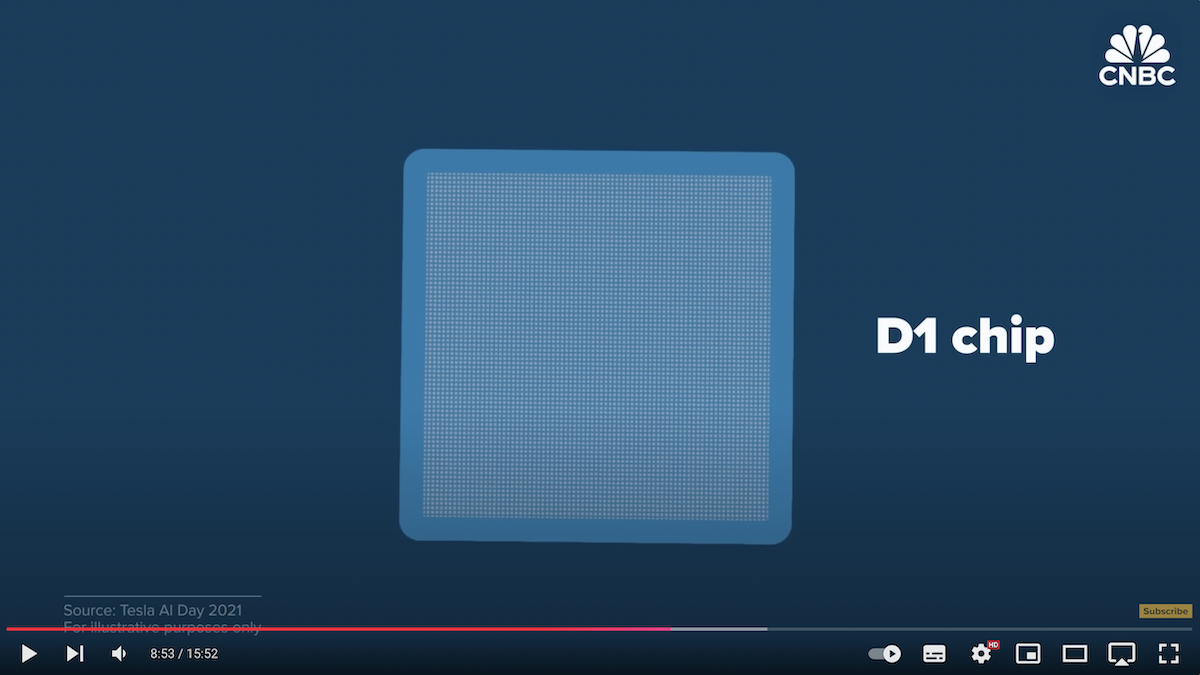
D2 Wafer
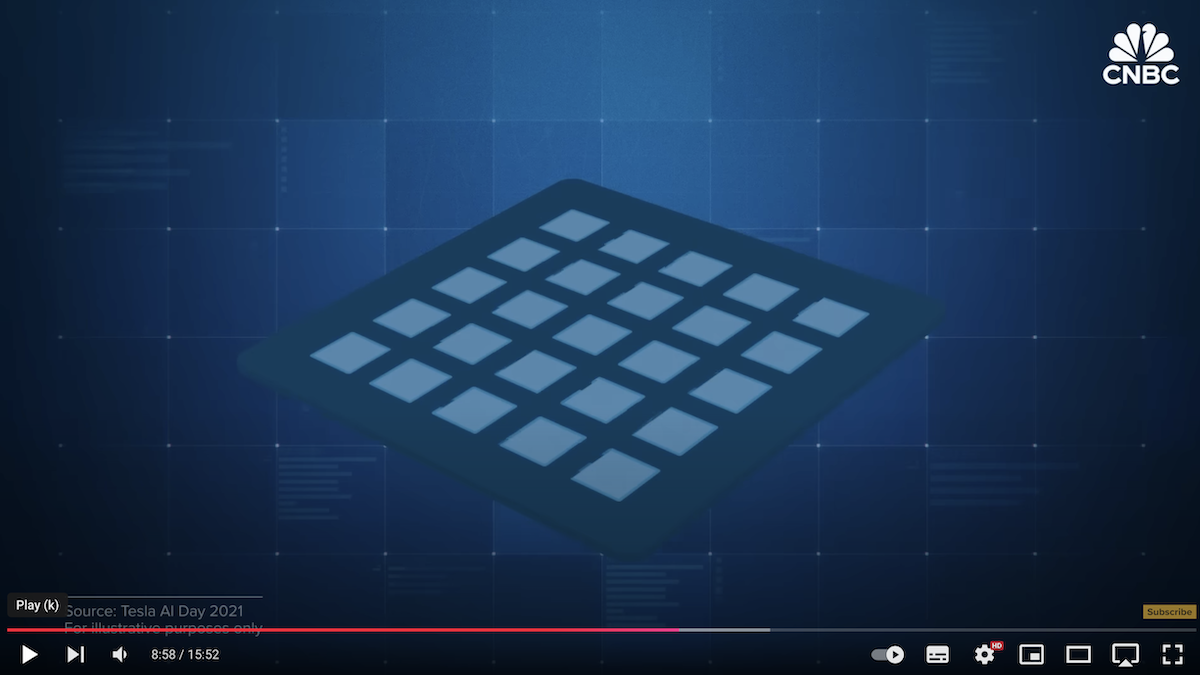
D2 Tile
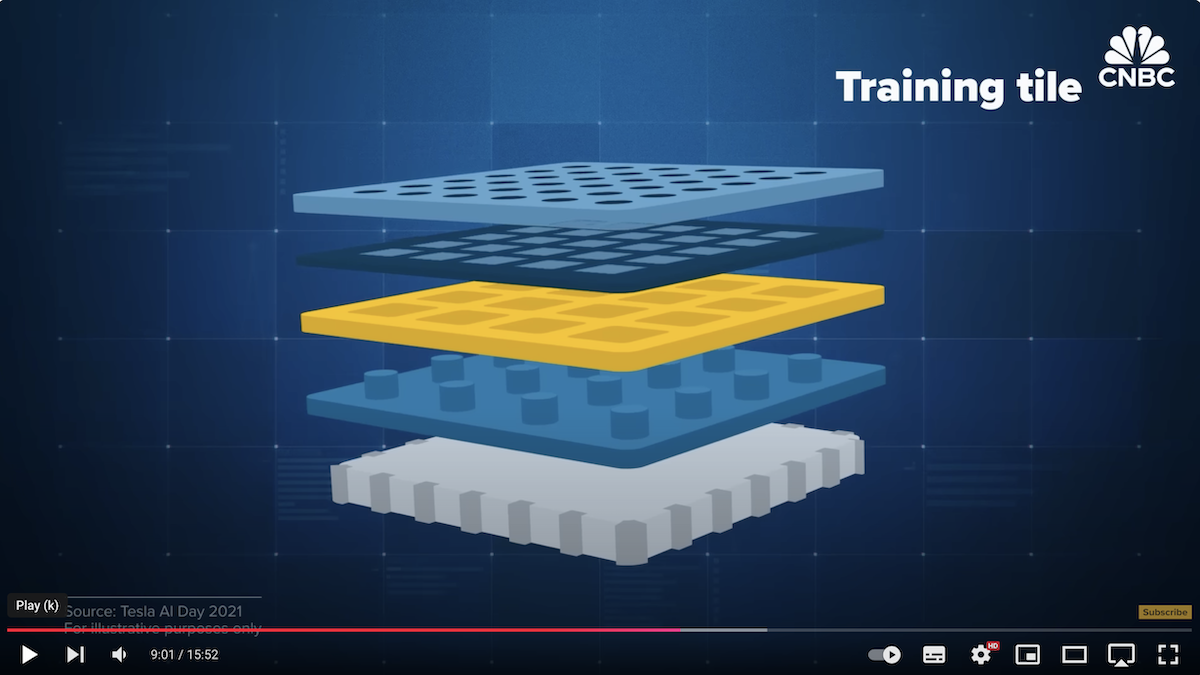
D2 Tray
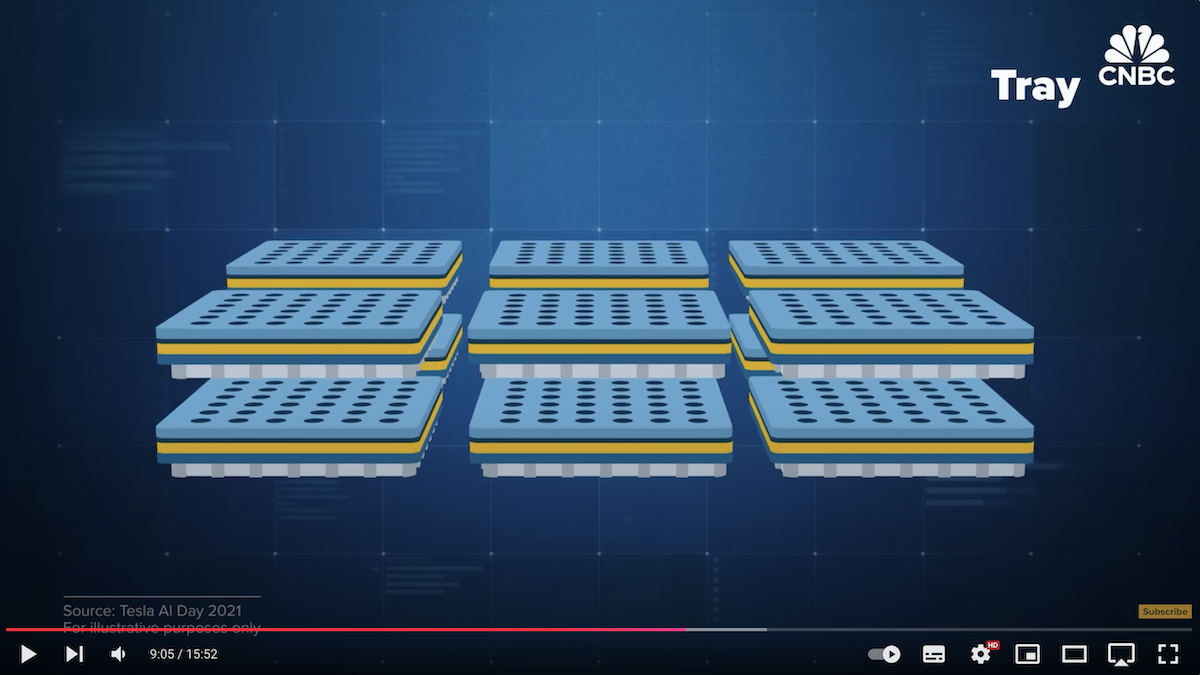
D2 Cabinet
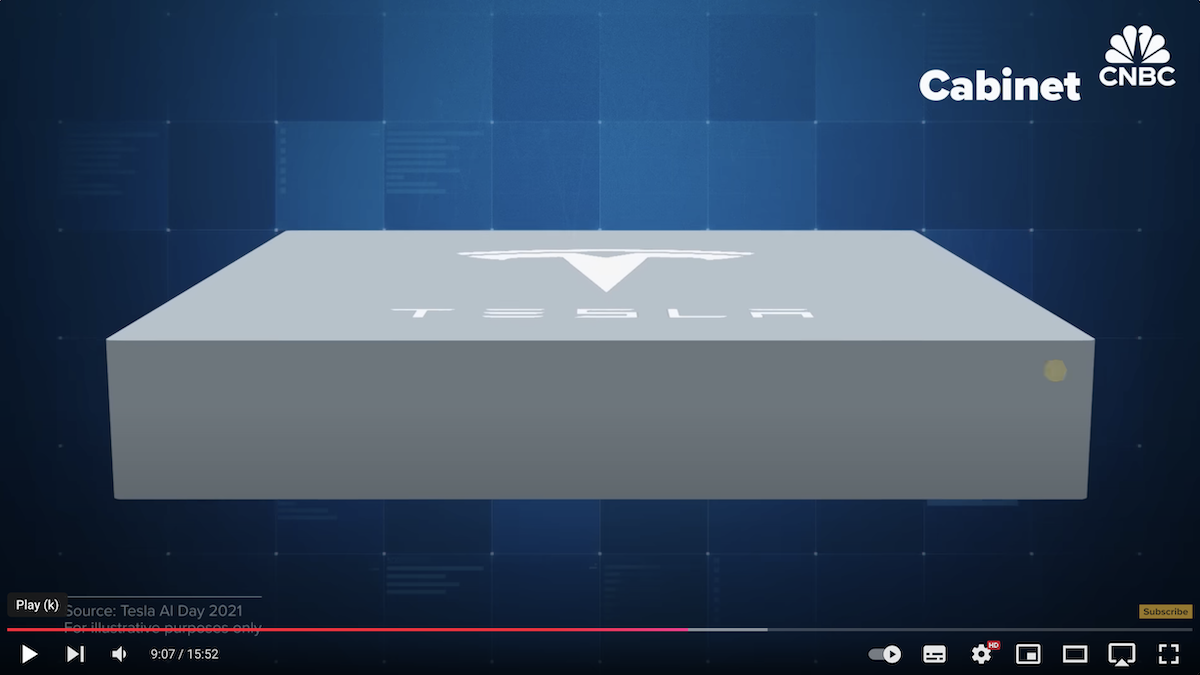
D2 Rack
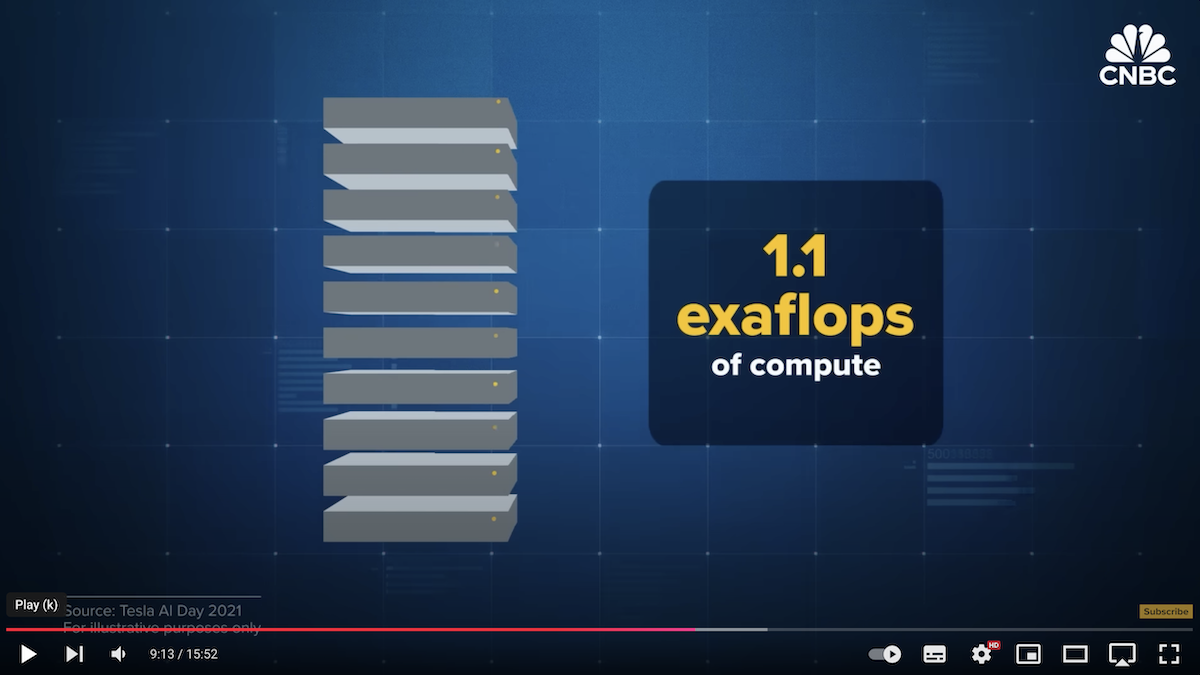