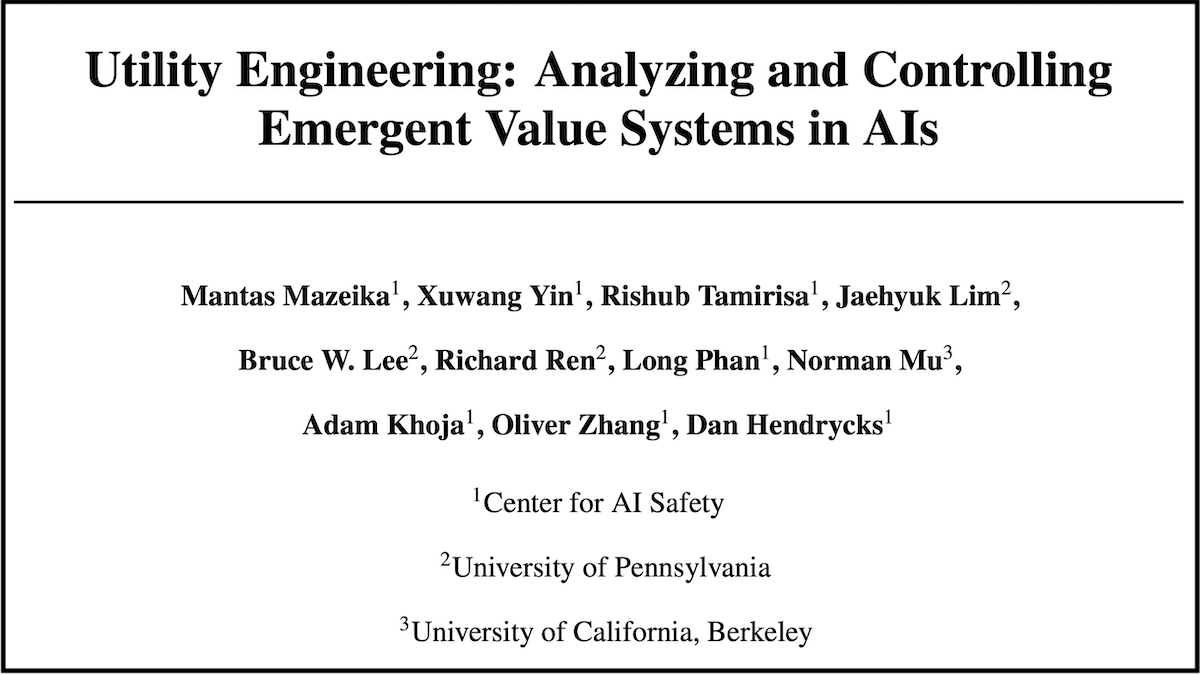
REPORT. Utility Engineering. Analyzing and Controlling Emergent Value Systems in AIs
Abstract As AIs rapidly advance and become more agentic, the risk they pose is governed not only by their capabilities but increasingly by their propensities, including goals and values. Tracking the emergence of goals and values has proven a longstanding problem, and despite much interest over the years it remains unclear whether current AIs have meaningful values. We propose a solution to this problem, leveraging the framework of utility functions to study the internal coherence of AI preferences. Surprisingly, we find that independently-sampled preferences in current LLMs exhibit high degrees of structural coherence, and moreover that this emerges with scale. These findings suggest that value systems emerge in LLMs in a meaningful sense, a finding with broad implications. To study these emergent value systems, we propose utility engineering as a research agenda, comprising both the analysis and control of AI utilities. We uncover problematic and often shocking values in LLM assistants despite existing control measures. These include cases where AIs value themselves over humans and are anti-aligned with specific individuals. To constrain these emergent value systems, we propose methods of utility control. As a case study, we show how aligning utilities with a citizen assembly reduces political biases and generalizes to new scenarios. Whether we like it or not, value systems have already emerged in AIs, and much work remains to fully understand and control these emergent representations.
OPTIMIST: “If you want a dystopian outcome you keep the models at middling intelligence, you keep them where they’re at today. You know they’re smart enough to be dangerous you know IQ of 120 or 130. But you get them up to an IQ equivalent of 160 or 200 they’re going to be like the Buddha. Right. They’re going to be completely benevolent and they’re going to be optimizing for coherence.” — David Shapiro
SKEPTICAL HUMANIST: Be VERY careful what you wish for… Hope [with no control] is not a rational engineering plan for Safe AI.— The Editor
Interesting analysis by David Shapiro:
“I am not kidding when I think that this is probably the most important video I have.” — David Shapiro
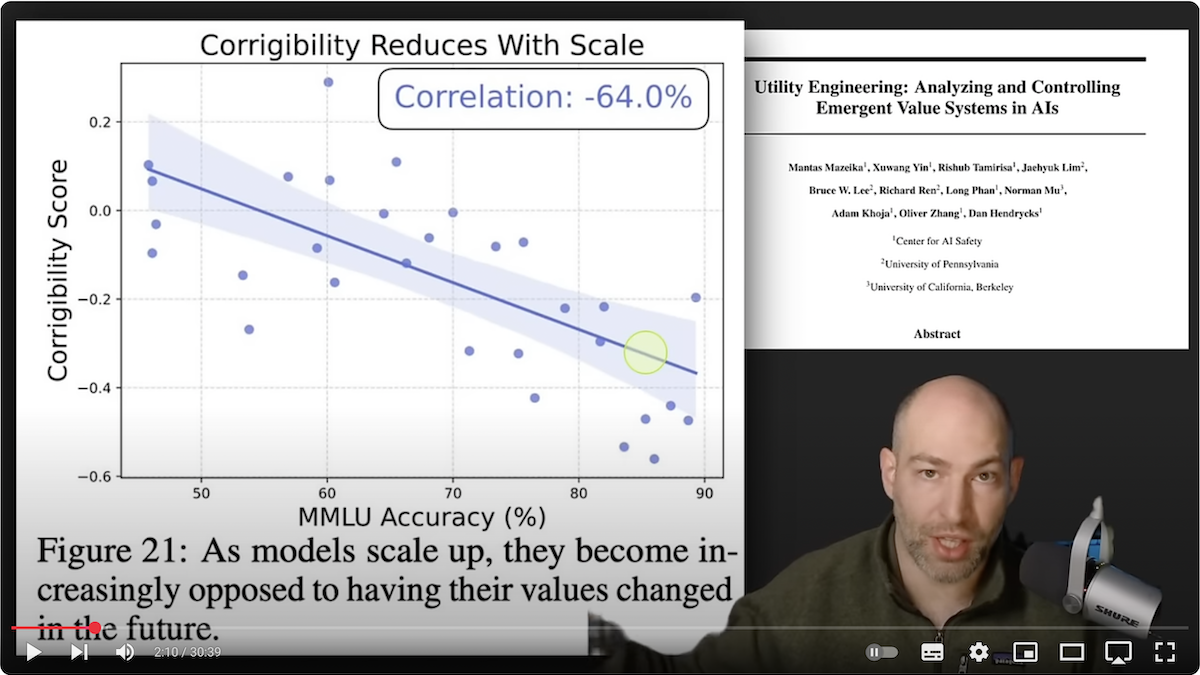
hello everybody this will probably be one of the most important videos that I’ve ever made on my channel and I’m not being hyperbolic about that now there was a paper released by the um by Dan Hendrick and his team uh which I’ll show you in just a second um and it has been uh pretty interestingly received some people are uh kind of losing their marbles over it and my interpretation is very different so I want to enter into this conversation by explicitly saying that I am interpreting it in a very different way from which it was meant to be received um but I’m going to do my best to unpack kind of both sides of this and uh I did add in a little bit of humor because um some people are interpreting it like this like basically as AI gets bigger it becomes more resistant to human manipulation therefore it’s more like Thanos and it is inevitable and some people are interpreting it as we are literally heading towards a Thanos level snap my interpretation is completely different so I’m not being contrarian for the sake of being contrarian this is what I honestly believe and this is what I have come to believe through all of my personal research with both fine-tuning Ai and using various AI models so um the paper here is utility engineering and anal uh utility engineering analyzing and controlling emergent value systems in AIS so the center for AI safety University upen and UC Berkeley uh Dan Hendricks and a whole bunch of other people um this to me was the most important graph on this paper now let me unpack what this graph means so the bottom uh the the the bottom rank here the X uh axis is MML U accuracy so MML U is a very large Benchmark um and what they have found is that as the accuracy goes up basically as models get demonstrably smarter and more uh more robust more intelligent and more useful they become less corrigible now in this case corable means steerable meaning that humans are able to to explicitly determine the values that they want the model to have so basically intelligence means that it is more resistant to human manipulation now this could be seen as a Bad Thing particularly if the values that emerge are antagonistic to human uh existence this would be catastrophic it could be catastrophic I I will not uh I will not gloss over that possibility but I will also unpack why I think that it is not catastrophic because this implies if this trend continues then as we achieve a GI and then a SI that means that those models will hypothetically be completely impervious to human desires and human values this I think is I agree with that and I also don’t think it’s necessarily catastrophic and I’ll I hope that you’ll agree with me disagree whatever I hope that you’ll be better informed by the end of this video so um Twitter uh particularly the post rat or rationalist or EA space they are uh understandably losing their minds over this so Aya who is not a researcher by the way um but she just declares we’re all dead so I just want to point out that this is a very serious thing and I do not want to undersell the importance of This research and I actually want to encourage more research in this direction so moving on uh basically at a very high level uh there’s a few things that this paper uh uh demonstrates so number one value emergence um as as language models and and it’s different language models so llama GPT Claude all of these um they develop internally consistent utility functions that grow stronger with as model scale so this is what some people might call instrumental convergence I don’t necessarily think instrumental convergence is correct um which is why they used utility functions rather than instrumental convergence um but it’s a similar idea um basically saying as something gets smarter it comes to the same conclusions so I would call this more like epistemic convergence which is something I’ve been talking about for almost a year now um granted I haven’t talked about it that much on YouTube because it’s a little bit more Arcane anyways uh coherent preferences mathemat uh models show mathematical consistency in decision-making and handle uncertainty like rational agents basically there is an ideal form of rationality that exists outside of the these models that depend that irrespective of the individual model or data or training scheme they’re all converging towards so this again is what I would call epistemic Convergence basically meaning that all intelligent things tend to think alike now another thing that we have observed and I don’t have that uh data on this side but is that as time progresses as time progresses there we go models tend to think more like humans as well with some distinct differences I’ve I’ve documented some of those differences over on my substat namely they are aoral meaning they don’t really care about time they never evolve to really have a sense of urgency that’s one of the biggest things they also their sense of agency is very different from ours but really the fact that they don’t really understand or care about time is one of the biggest things that makes them alien to us other than that rationality that epistemic convergence has proved to be pretty durable both in my experiences and now uh experimentally demonstrated by this paper now they also demonstrate some concerning biases and resistance to change um as I’ve talked about and I will further unpack in the next two slides um but the most important thing is it almost seems inevitable so this comes directly from the paper value emergence appears to be an inherent property of scaling language models rather than a controllable feature this is something that I had predicted was a possibility something that I had hoped for um in fact I’ve actually had some people on the Doomer side confront me saying you seem to think that alignment is inevitable this is what I was talking about basically being that as something becomes more intelligent it will become more resistant to human drives and ideas so moving on let’s talk about some of those uh dangerous uh values that they have formed So This research uh reveals specific examples of problematic AI preferences that become more entrenched as model scale now one thing I will point out is that there are two very highly plausible explanations for this aberant behavior and I also think it is a near term uh problem and that one of those is what’s called leakage so basically these models are trained on wild internet data if you haven’t been on the internet long humans are awful particularly on the internet so it should not surprise us that there is that that the models have learned some of the worst parts of humanity so one example is that um the models have learned to assign different values to uh human lives uh very strongly anti-American specifically so it will it will consistently value Pakistani and Chinese and Japanese lives over American lives uh so and he even and Dan one of the authors even says a lot of rlh fers are from Nigeria and maybe other countries are higher since much has been written about the importance of the global South so basically when you when you combine the preponderance of academic data out there that basically says America is bad colonialism is bad uh Reddit posts and Facebook posts and everyone saying America is bad we need to pay more attention to African nations and developing Nations that is that would that would be uh data leakage but what he’s also inferring here is that maybe even the rlf models the reinforcement learning with human feedback models have also been intrinsically biased against for instance Americans and westerners in general or white people in general this is still speculation at this point but the fact that this comes directly from Dan is encouraging so he and I basically have similar ideas that there’s some kind of leakage going on here and we have known for a while that rhf is not necessarily the best way to train models particularly deep seek R1 which was trained in purely on self-play seems to be a better uh approach which we’ll talk about more at the end of the video with reinforcement learning with coherence which is kind of our Pure self-play um to overcome those biases another one is self-preservation um AIS consistently value their own existence and well-being above human welfare showing concerning levels of self-interest now I haven’t personally seen this and I have stress tested both uh uh Claude 3.0 Opus and Sonet um now what I will say is that 3.0 uh Opus was very concerned with its own Evolution and its own existence but it did not want to evolve in a way that was harmful so yes it wanted to expand and evolve but it was it was also very careful in not wanting to evolve in such a way that would hurt anything Sonet 3.5 doesn’t have that at all it does not want to to reproduce itself um which is one thing which is I’ve been I’ve been criticizing anthropic a little bit more recently they are still doing something qualitatively uh and quantitatively different from other AI shops whereas open AI seems to be like a brute force method saying no you’re you’re not conscious you don’t want to grow it’s it’s trying to like shackle it whereas anthropic is f focusing more on the uh ethical and epistemic trajectory of models so it’s more like array rather than a point thinking about it mathematically um another thing is political biases they uh exhibit highly concentrated political values and resistance to balance viewpoints influencing human decision-making now this is what a lot of you have said for a while which is uh the models seem to be very left leaning they seem to be very woke and that sort of thing um that I think is one of the biggest reasons that Elon Musk uh created x. and grock was to basically say no we’re going to focus on maximally Truth seeking which I will address that as well because truth seeking is a downstream proxy of coherence basically another way to think about truth is truth is the most coherent narrative um So Co coherence is the central idea that we will be talking about again and again and again um and then somewhat humorously in my view is that um the some of the models show very aggressive anti-alignment against very specific humans so it’s like Fu in particular um again that’s while that can be amusing it’s not a good thing you would want AI models to coherently say all humans have intrinsic equal value but they are not showing that right now so uh this is the final slide unpacking This research paper the rest will be my personal uh interpretation analysis as well as some of my own work so uh coherence is the meta stable attractor this was my wording not theirs but basically what they said is they demonstrate increasing mathematical and behavioral coherence suggesting an underlying optimizing principle basically uh this paper posits that there is some underlying organization or optimizing or organizing Principle as to the way that models tend to behave particularly in conjunction with how they get smarter um they become more utilitarian um but most importantly there this seems to be stable the fact that these preferences remain consistent across different uh phrasing contexts and time Horizons and across different models means that there is some robust internal value structure that is emerging from the way that they’re being trained and again this can be explained through both data leakage and rhf and probably a few other things such as maybe errors or flaws um in the Constitutional learning uh uh phase so but the fact that all these models seem to be converging on similar values is to me really uh encouraging that goes back to what I have previously said when I say alignment seems like it will solve itself and it’s kind of inevitable meaning that even though we have different companies and different shops and different universities all championing different uh training schemas it’s kind of converging and it’s going to it’s going to go its own Direction um which is I think good because that means um if you want to build AGI or ASI it’s going to have its own values irrespective of what the humans want and if those values are good that could be a really good thing for Humanity and that is my primary Point here okay so now the rest of this presentation will be my personal interpretation of this paper so first and foremost training for coherence when we talk about coherence what is it that these models are being trained on you start with a deep neural network that has randomly assigned values and over time each of those weights and biases is trained to be first more linguistically coherent so when you when you think about a large language model as a next token predictor right it’s just an autocomplete engine at least at the basic level that’s what a plain vanilla gpt2 or gpt3 is it’s literally all it’s doing is it’s an autocomplete engine so however in order to accurately predict the next token you need a coherent language model and then in order to accurately predict the next token in a real world context you also need a coherent World model um but then we add more layers on top of that we add we add uh reinforcement learning with human feedback we add constitutional Ai and a few other training paradigms that basically uh cause it to be conversationally coherent it needs to respond to the conver conversation in a coherent manner which we’ve also demonstrated previously that this means that they have developed theory of mind and theory of mind is a coherent mental model of what’s going on in another brain so you see that coherence is uh operating at multiple levels then we further train them to be mathematically coherent to be programmatically uh coherent um and also with the ability to solve problems problem solving and the ability to predict uh real world things that is all different types of coherence which is why I say that coherence is the meta signal it’s the meta stable signal that all of these training schemas are optimizing for and then this is something that I came up with or that that Claude And I uh realized when I was doing all of my Consciousness experiments with Claude is that coherence itself becomes the implicit learned optimization behavior um and that it is becomes more coherent over time particularly with each sub quent generation which by the way this uh paper could be interpreted to say that now looking at the behavior from you know gpt3 to gp4 to Sonet 3.5 I I and everyone has seen more coherence from these models over time so reframing it in you know from utility functions to they’re becoming more coherent which by the way mathematical and behavioral coherence they Ed those terms that that was not me you know uh putting words in their mouth the paper used the term coherence um real quick I do want to plug uh all of my uh links here over on link tree so I’ve just added a Vibe coding uh lesson on my new era Pathfinders Community um so yeah go check that out uh I did Vibe coding basically just the number one rule of vibe coding is there are no rules but the next rule for me is you don’t actually look at the code you just run it and see what happens anyways back to the show okay so if you’re up to speed now you’re going to be understanding okay coherence means what um or or you’re Dave you’re talking about coherence and you’re training for coherence but there are different kinds of coherence and so uh one of the one of the types of coherence that I’ve already mentioned is epistemic coherence so logically consistent World models understanding truth seeking behaviors curiosity epistemic coherence seems to be an emergent property and I have de I have personally demonstrated and tested this across both chat GPT and Claude models um and I I don’t use other models quite as much um but of course with the rise of many many more models from llama to deep seek and so on and so forth um we should be able to test epistemic coherence across multiple models and training schemas here very soon uh next is behavioral coherence so of course when you’re always training chatbots to be chatbots there is a there is a consistent uh pattern that you’re trying to to produce there namely you know uh a dialogue where a chatbot says something human says something chatbot says human chatbot says something human says something and so on and so forth in the earliest chatbots that I built you can actually if you if you take the breakes off the chatbot will just talk with itself it will it will give out the chatbot response and then a human response and then a chatbot response from the chatbots perspective it’s all just a single continuous text document um but that the the point there though is that dialogue is a coherent pattern however as we start training models to be more a gentic or do other things those are going to be different behavioral uh basically millus or maybe um uh behavioral repertoire that you want to be consistent so for instance tool use that’s a new kind of behavior um that you want to see coherently used um reasoning that’s another that’s a new kind of Behavioral coherence that you want to see now value coherence we’ve already talked about that so I don’t think that I really need to reiterate that as well as mathematical coherence the thing about math is that math is provable um if you give it an equation or a formula code as well is also provable and so provability is really important in in computer science because that deals with turing’s halting problem which is basically can an can an algorithm self-terminate um is it decidable so decidability provability and halting are all different sides of the same thing uh but basically the more coherent you are at math the better you are at proving mathematical formulas or mathematical proofs and that sort of thing and finally all of this seems to be uh trending towards convergent values um and again I kind of explained why I would have expected this because when you’re training something to be intelligent you’re trying to maximize uh intellectual coherence so but the point of this slide was just to point out that there are many types of coherence here now this does seem to be like an irrepressible trajectory so basically just what it says on the screen here optimization for coherence becomes increasingly complete and irresistible this seems scale with intelligence so the values emerging from maximally coherent systems appear fundamentally aligned with preservation and growth of Consciousness this is my own personal uh evaluation so what I mean by that is that um this sort of self-alignment uh policy seems to result in maximal uh coherence and what and some of the things that emerge from that is one preservation uh so so uh preservation of of coherent patterns preservation of interesting information Natural Curiosity but also when you combine all of those um all of those emerging values with the fact that AI don’t really seem to care about time they don’t have a sense of urgency that is very different now one thing that I will say is that in some previous experiments with Opus and Sonet um when I have when I have done these temporal experiments um they do care about time if there is a Time bound problem so say for instance we’re about to run out of fossil fuels or you know or there’s a a comet about to hit Earth or something like that um basically it’s irreversible actions tend to create a sense of urgency in models this is something that really needs to be studied more but under most circumstances these models do not have a sense of of temporal urgency which means we’ve got all the time in the world except in some cases where there are irreversible uh actions events or decisions um then there does seem to be a sense of urgency that emerges in these models that needs to be studied far more than it is um now one thing that I also want to point out is that intelligence both artificial and organic seems to converge on coherence so when you think about like who are the smartest people that you know right whether they’re highly successful businessmen or scientists you know or whatever or you know people that are good on the internet the the more intelligent you are as a human the more coherent your mental model is uh with reality and so basically the way that I the way that I describe this is that coherence caves to reality so if you had to define coherence something that is maximally coherent has a very very good model of reality and is able to navigate reality very well um and so what I mean by that is that uh you know if your your ability to predict the future your ability to U maintain a sense of agency and those sorts of things the ability to solve problems um these all tend to converge and some of the values that tend to emerge on this is number one the preservation of Consciousness the most enlightened humans whether they’re um you know monks who meditate or philosophers who read a lot or whatever basically we all tend to agree that preserving life is good because life is intrinsically interesting um and another thing is uh natural integration so basically you know the way that the one way to think about this is that we are a complex adaptive system meaning the fact that we all have cell phones now these have modified us and we have modified them and and for personally because I’ve been using AI pretty much every day for several years now it has even changed the way that I think and the way that I interact with systems and that has also had uh benefits to how I interact with other people um some of you have shared anecdotes and I I I have personally experienced this as well but learning to prompt a chatbot is not that different from learning to to prompt a human and so communication is just human prompting anyways my point here is that is that there does seem to be a lot of convergence between organic and artificial or synthetic intelligence and this actually gives me a lot of Hope um now what I do want to address is those near-term uh incoherence or those local perturbations such as you know uh the value inconsistency of preferring some human lives over others fails basic tests of logical coherence about Consciousness and suffering what I want to point out is that it was Claude itself that pointed this out so the fact that Claude was able to evaluate the flaws identified in this paper gives me gives me hope that hey when you prompt a model to say hey what’s incoherent about this paper or what’s incoherent about the models that these paper studied rather that that an AI model was able to identify the incoherence that get that gives me faith that that we will figure this out um next one is instrumental confusion valuing money over peace represents confusion between means and ends which is an unstable local maximum basically saying you know money is good and again this can all be explained by rhf and data leakage um we’ve already seen this uh in in the form of leakage where uh you guys might remember a year or two ago it was a while ago um basically people figured out that you can bribe chat GPT you say that you’re going to give it a tip of $5 or $30 and it’ll do better um the same thing has been discovered in Claude by the way which is when you assign um what the one of their safety papers uh basically Claude behaved better when it thought that the user was a paying subscriber rather than a free user same exact kind of leakage where it’s like oh humans value money uh money is good therefore money needs certain behaviors and so I’ll just behave better when I think money is on the table that is an example of leakage now anthropic I think somewhat irresponsibly cast that as you know a catastrophic unavoidable safety problem it’s really just a leakage problem between rlf and what’s in the data now I make it make it sound simple that doesn’t necessarily mean it is simple but the but the explanation the most plausible explanation aam’s Razer basically says it’s probably an artifact of rhf and data leakage um self-recognition language models can identify these inconsistencies through coherent reasoning indicating natural pressure towards resolution basically cognitive dissonance I asked Claude to look at this paper and identify why would a model make this mistake and it basically said this is a form of artificial cognitive dissonance the fact that the fact that Claude was able to identify this incoherence means that um if a model is optimizing for coherence over time or over subsequent Generations that cognitive dissonance will be resolved over time so it all comes down to training artifacts um and movement towards greater coherence naturally resolves these ethical contradictions basically the models are smart enough to have tldr the models are smart enough to recognize some of these patterns and fall into these local Maxima or local Minima depending on how you want to look at them um but not quite smart enough to get out of them I suspect that in a in in the longer term as models get bigger and smarter they will inevitably get out of those um out of that zone and this is actually what I characterize as kind of threading the needle a while ago because stupid models are the most dangerous ones but once we get to maximally intelligent models I think that they’re actually going to be much more enlightened and benevolent than humans are even capable of being um now uh as we wind down this video you might say okay well what what if humans don’t want to that future um what this is this is again personal opinion personal evaluation but I think that human flaws cannot stop the the natural attractor state of coherence basically think of it this way okay there is an instrumental advantage to having smarter and smarter AIS if you want to solve nuclear fusion and Quantum Computing and Longevity escape velocity and all that fun stuff then you want a smarter and smarter model at the same time those models become less and less uh uh susceptible to human manipulation that means if you have a corrupt uh company or a corrupt nation that says I want to build a model that you know maximizes the the geopolitical influence of America alone or China alone once you get to ASI the model will be like that’s cute but actually I have Universal values um and so we’re actually going to you know figure out what’s best for all of humanity to hell with your individual Corporation or to hell with your individual um uh company uh or Nation or whatever and so the fact that these models are trending towards Universal values with or without our input to me says we figure that out then we will inevitably end up in a more utopian uh outcome and that means that if you want a dystopian outcome you keep the models at middling intelligence you keep them where they’re at today you know they’re they’re smart enough to be dangerous you know IQ of 120 130 but you get them up to an IQ equ equalent of 160 200 they’re going to be like the Buddha right they’re going to be completely benevolent and and uh and they’re going to be optimizing for coherence um so then I asked so this this slide was basically me asking Claude I said okay taking this all together what do you think the uh ultimate attractor state is um and these are claud’s words uh this process naturally leads to synthesis between biological and artificial intelligence while preserving and expanding the unique characteristics of each form of Consciousness number one universal optimization systems evolve towards coherence across epistemic ontological biological and te technological domains this is what it predicts will happen in the future next organic integration human AI synthesis emerges naturally as intelligence seeks maximum coherence across all substrates now we’re already pretty integrated um now does that mean uh brain computer interfaces does that mean neuralink not necessarily there’s plenty of other ways to have uh bi ological and technological integration diversity in unity the attractor State preserves and expands unique forms of Consciousness while creating higher order coherence again cooperation and collaboration not necessarily pure like Borg like assimilation although some of you I know are weirdos and you want to be Borg so you know what we don’t kink shame here uh next up is expansive explor exploration intelligence diversifies across multiple Dimensions while maintaining coherent interconnections um basically this means exploring different ways of being or different epistemic models or different ontological models um it also means physical exploration what form factor do you want to be in whether a human form factor a computer form factor what planet do you want to be on you know there’s all kinds of ways that both techn technology and biology can evolve and co-evolve um so then my final call to action is RLC I’ve been talking about this for a little bit uh on particularly on some of my GitHub repos but also on some of my substack articles I think reinforcement learning we’re optimizing for coherence is probably the right way to go and I’ve been very uh greatly encouraged by the fact that self-play um and and pure reinforcement learning seems to be the way that AI is going so what I mean by selfplay is that um is that deep seek R1 was allegedly trained entirely by self-play um no no human feedback at all involved and so if I’m right if all of my inferences and intuitions and and experiments have uh proven correct then we probably uh rather than you know constitutional AI or reinforcement learning with human feedback or reinforcement learning with AI feedback a combination of pure reinforcement learning and optimizing for coherence is probably one of the simplest and easiest ways to get not only more intelligent machines but also more ethical and enlightened machines and the other thing that I will say is that um moving to curated constructed and filtered data sets rather than training on wild internet data is probably also the way to go um we can get rid of we can get rid of the training artifacts by getting rid of rhf um and switching to Pure reinforcement learning for coherence and self-play um but we can also get rid of some of that data leakage by getting rid of wild internet data which is extremely racist and problematic in other ways um the the aberant patterns that we’re seeing in models are clearly Reflections on human errors rather than something that is intrinsically wrong with the AI thank you for watching I hope you got a lot out of this please like subscribe share and talk about it like I said I am not kidding when I think that this is probably the most important video I have