U.S. NATIONAL CANCER INSTITUTE. Artificial Intelligence (AI) and Cancer
AI presents an unprecedented opportunity to advance our understanding of cancer and improve care for people with cancer.
Artificial intelligence (AI) is a machine’s ability to perform functions that are usually thought of as intelligent human behaviors, such as learning, reasoning, and solving problems. Computers derive this ability from algorithms that enable the use of data to make predictions or to create new content. AI algorithms can detect patterns in large amounts of data and identify relationships among pieces of data that cannot be easily perceived by the human brain.
In recent years, advances in three areas—methods and algorithms for training AI models, computer hardware needed to train these models, and access to large volumes of cancer data such as imaging, genomics, and clinical data—have converged, leading to promising new applications of AI in cancer research.
These new applications include understanding and predicting biological mechanisms, finding and leveraging patterns in clinical data to improve patient outcomes, and disentangling complex epidemiological, behavioral, and real-world data. Implemented in an ethical and scientifically rigorous manner, these uses of AI have the potential to rapidly advance cancer research and create better health outcomes for all.
AI applications in cancer research and care
NCI research is advancing the use of AI across the spectrum of cancer research and care, including mechanisms of cancer, cancer screening and diagnosis, drug discovery, cancer surveillance, and health care delivery.
Advancing fundamental knowledge of cancer biology
AI methods are being applied to advance knowledge about mechanisms of cancer initiation, progression, and metastasis. For example:
- The body of scientific literature provides a vast resource of information and knowledge on cancer. Artificial intelligence experts are taking advantage of large language models to develop new computational tools to improve knowledge extraction from research publications.
- As part of the collaboration between NCI and the Department of Energy, researchers are using AI to simulate the atomic behavior of the RAS protein, one of the most commonly mutated proteins in cancer. A better understanding of how RAS interacts with other proteins could help scientists find new avenues to target cancer-causing mutations in the RAS gene.
Expediting cancer screening, detection, and diagnosis
AI is helping to improve the speed, accuracy, and reliability of some cancer screening and detection methods. For example:
- The Food and Drug Administration has authorized the marketing of AI-based software to help pathologists identify areas of prostate biopsy images that may contain cancer.
- Medical images such as mammograms can be rapidly processed with the help of AI, allowing radiologists to focus their time on other tasks that require their technical judgement. NCI-supported research has shown that AI imaging algorithms not only improve breast cancer detection on mammography but can also help predict long-term risk of invasive breast cancers.
- NCI scientists are using AI to improve cervical and prostate cancer screening. One group of NCI researchers and their collaborators developed a deep learning approach for the automated detection of precancerous cervical lesions from digital images.
Accelerating cancer drug discovery
AI is being used in many ways to develop new treatments for cancer through novel approaches to drug discovery and design, drug repurposing, and predicting patient responses to treatment. For example:
- NCI researchers and their colleagues have employed AI to help predict how immune cells called T cells will respond to tumors. They used machine learning to find patterns within a large volume of human and mouse T-cell activation data and predict T-cell behavior with the aim of improving immunotherapies.
- New AI methods are helping researchers study the biological mechanisms of drug response. For example, NCI-supported researchers have developed predictive AI models that use deep learning to provide a map of common drug response pathways.
Facilitating precision cancer treatment
Learn More:
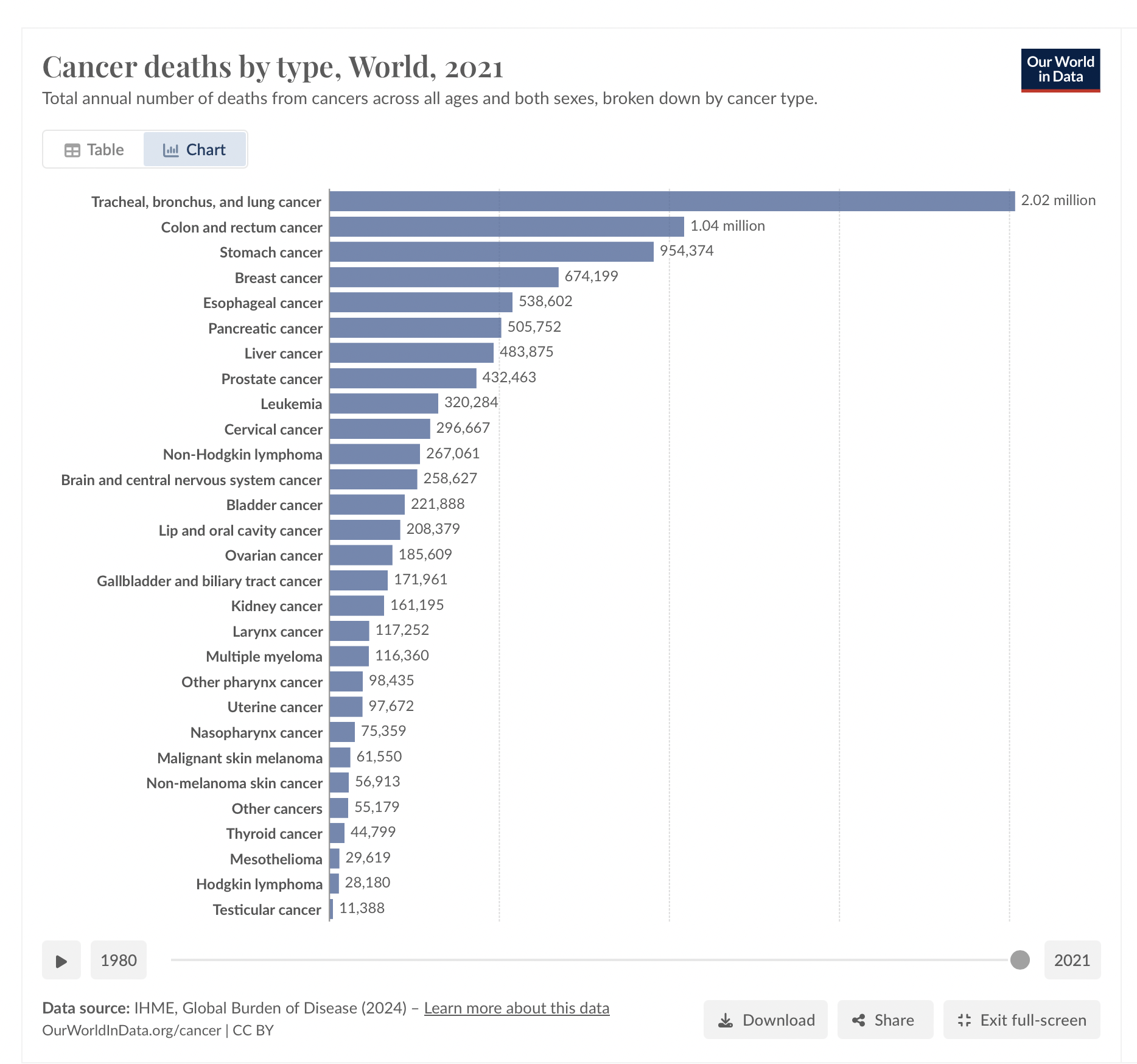
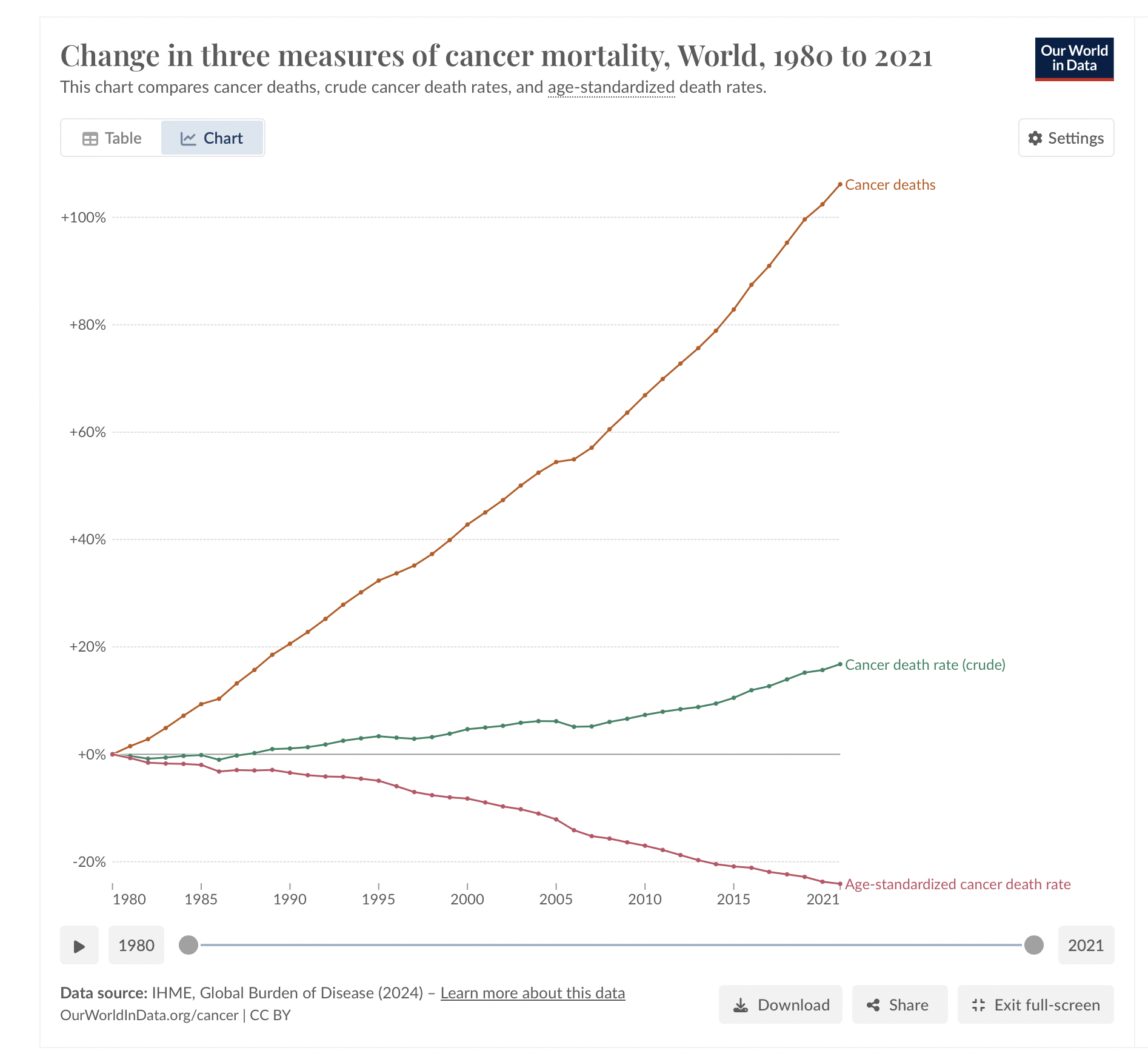