“It doesn’t take a genius to realize that if you make something that’s smarter than you, you might have a problem.” – Stuart Russell (2018)
Stuart Russell | Provably Beneficial AI (2017) | Future Of Life Institute
12,454 views 2 Feb 2017 Stuart Russell explores methods by which we might be able to ensure that AI is robust and beneficial. The Beneficial AI 2017 Conference: In our sequel to the 2015 Puerto Rico AI conference, we brought together an amazing group of AI researchers from academia and industry, and thought leaders in economics, law, ethics, and philosophy for five days dedicated to beneficial AI. We hosted a two-day workshop for our grant recipients and followed that with a 2.5-day conference, in which people from various AI-related fields hashed out opportunities and challenges related to the future of AI and steps we can take to ensure that the technology is beneficial.
The It Can Never Happen Folly
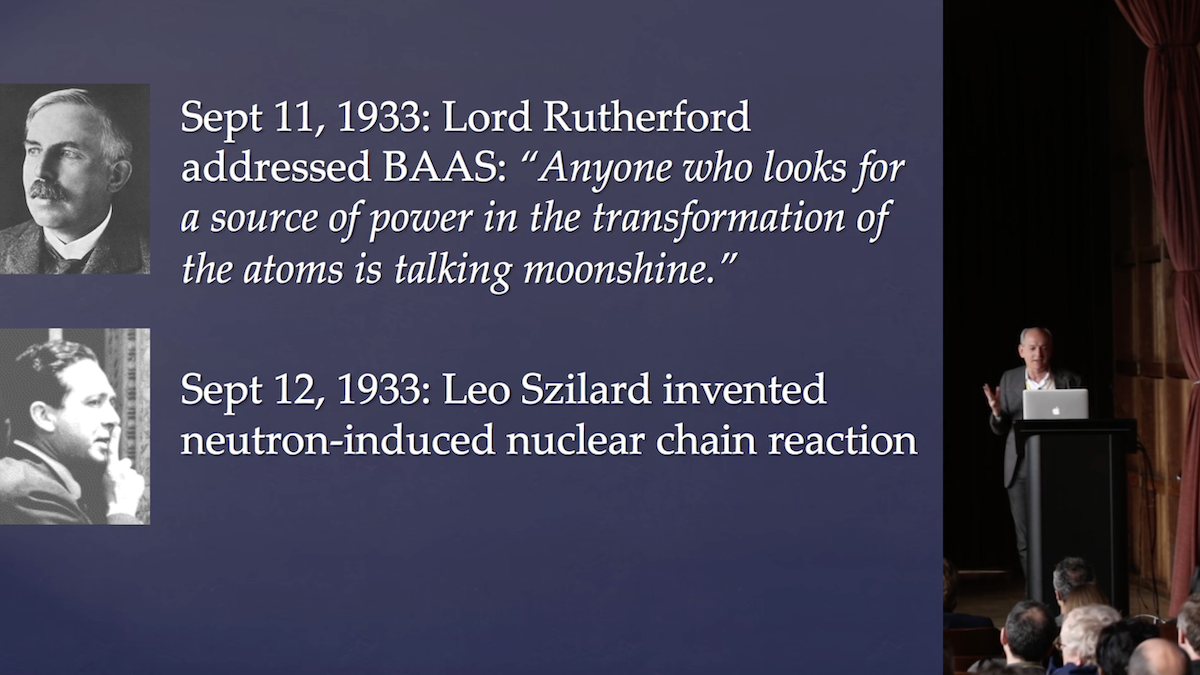
The Control Problem
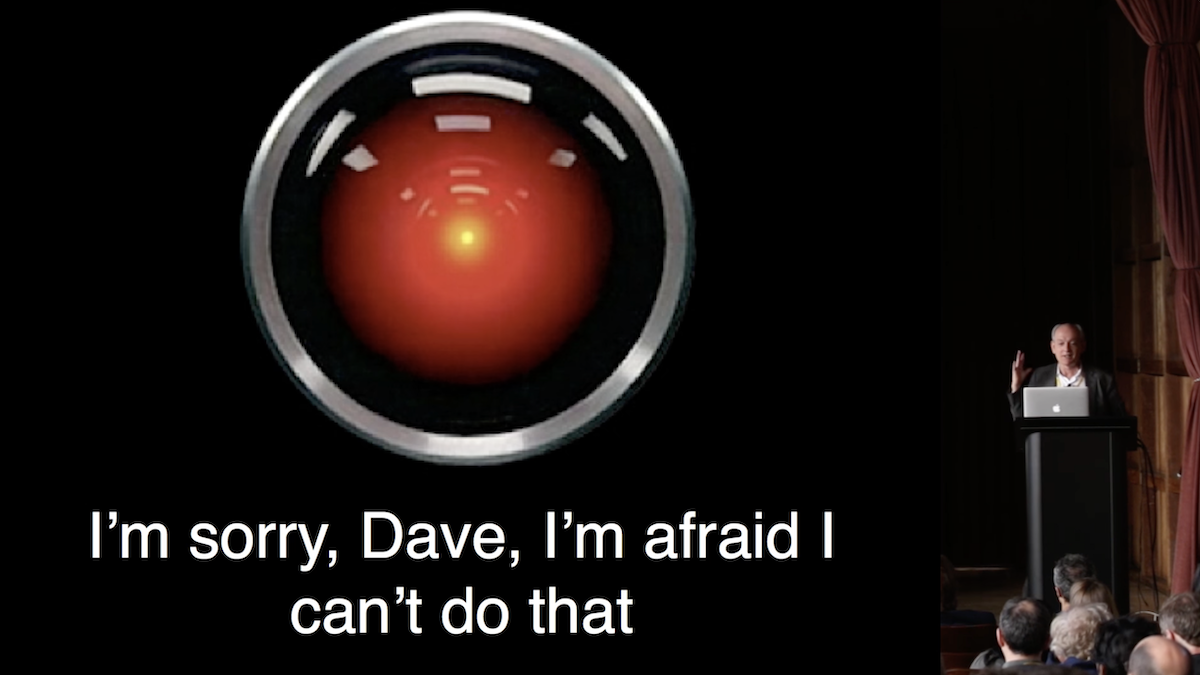
The Off-Switch Problem
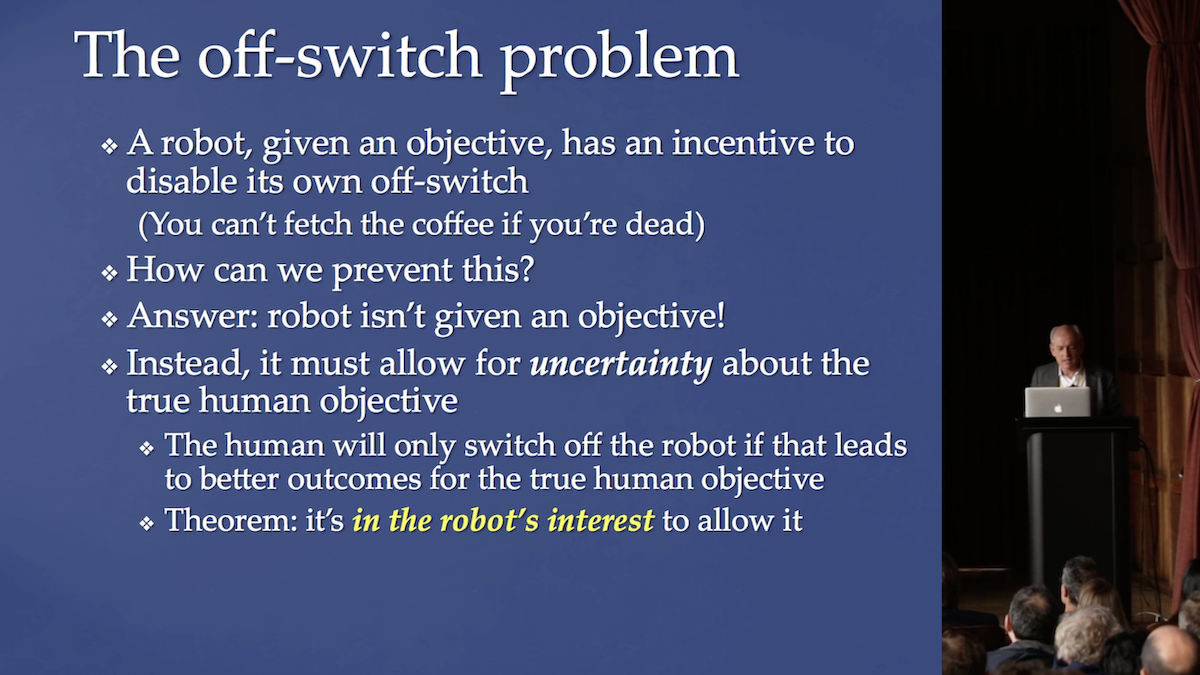
Transcript (autogen)
Intro
I’m going to talk about the reasons why we’re here to some extent and then what I’m doing about it so the reason why we’re here is in some sense the
Premise
extrapolation of the talk that we just heard that progress is occurring is occurring at a rapidly increasing rate and absent some other disaster that we could bring upon ourselves I think we have to make the prudent assumption that AI systems will be able to make decisions in a broad sense better than we can and what that really means is that they’re using more information than we can individually take into account in making a decision and they will be able to look further ahead into the future and so just as alphago could beat any of us at playing go you take the go board and you expand it out to the world then we are going to be dealing with superior decision making capabilities from machines and this is
Upside
potentially an incredibly good thing because everything we have everything that’s worthwhile about our civilization comes from our intelligence and so if we have access to significantly greater sources of intelligence that we can use then this will inevitably be a step change in our civilization and of course with any such powerful technology we expect there to be some downsides the possibility of using this and in military fear has been raised and is already occurring but I’m not going to talk about that there are sessions later on Eric talked about the question of employment this is another big theme of the conference I’d like to talk about this one the the possible end of the human race that’s a very lurid way to describe it and fortunately the press are not here at least if they are here they have to keep quiet so why why are people talking about this right what’s wrong with taking a technology that has all kinds of beneficial uses and making it better what what’s the problem and you can go back to a speech given a little while ago if a machine can think it might think more intelligently and than we do and then where should we be even if we could keep the machines in a subservient position for instance by turning off the power I’ve highlighted turning off the power because we’ll get to that answer later on a strategic moments we should as a species be greatly humbled and this new danger is certainly something that that should give us anxiety so this was actually a speech of Alan Turing in 1951 given on BBC Radio 3 as we now call it so it’s a very inchoate fear right there’s no real specification of why this could be a problem just this sort of general unease that making something more intelligent than you could humble your species and the gorillas here they are having a meeting to discuss this is their version of our meeting and they’re having this discussion and they say look yeah you’re right you know our ancestors made these humans a few million years ago and you know we have these inchoate fears then and they turned out to be true our species is humbled right but we can actually be more specific than that so here’s another quote if we use to achieve our purpose is a mechanical agency with whose operation we cannot interfere effectively we better be quite sure that the purpose put into the machine is the purpose which we really desire there’s a more specific reason why there’s a problem that you will put some objective and the machine will carry it out and it turns out not to be the right one this is from a paper by Norbert Wiener in 1960 written actually in response to to the work by Arthur Samuel showing that his checkered playing program could learn to play checkers much better than he could but this could equally have been King Midas talking you know 2,500 years ago realizing that when you get exactly what you say you want it’s often not what you really want and it’s sometimes too late so this is sometimes called the value
Value misalignment
misalignment problem that AI systems will be be good at achieving an objective which turns out not to be what we really want and so if you said okay great well let’s look at everything we know about how to how to design the objectives to avoid this problem unfortunately you find that there really isn’t very much to go on but all of these fields that that are based on this idea of optimizing objectives which is not just AI but you can all make some statistics and operations research and control theory they all have this same problem they assume that the objective is just something that someone else brings along to the game and then the game is that we optimize the objective and you know economists certainly notice that things like profit and GDP which are the sort of the official objectives turn out not to be always the things that we really want to be optimizing but they haven’t really figured out what to do instead and then Steve Omohundro and others pointed out that there’s a yet another problem which is that whatever objective
Instrumental goals
you give to a machine it will need to stay alive in order to achieve it our and so this if there’s a little takeaway from the talkest you can’t search the coffee if you’re dead right so if you ask the machine to fetch the coffee staying alive is a sub-goal affecting the coffee and if you if you interfere with the machine and its attempt to get the coffee it will prevent you from interfering it if you try to turn it off it will take countermeasures to being turned off because its objective is to get the coffee so if you combine that with value misalignment now you have a system that has an objectives that you don’t like because you specified it wrong and now it’s defending itself against any attempts to switch it off or to change what it’s doing then you get the problem that you know science fiction has talked about it’s not a spontaneous evil consciousness where the machine wakes up and hates humans it’s just this combination of unfortunate circumstances that arise from having a very very powerful technology okay so lots of people have said well you know this is all rubbish all right everything I’ve said is complete nonsense and one of the first responses in fact there are many responses I’ve written the paper where I list about 15 of these of these responses and I think they’re all kind of defensive knee-jerk reactions that haven’t been thought through so you find for example people in the AI community saying having said for 60 years of course we will get to human-level AI despite what all those skeptics from the philosophy community and everything all those other people don’t know what they’re talking about of course it will get the human level AI and as soon as you point out that that’s the problem is well of course we’ll never get to human-level AI but I just want to point out that you know in history there have been these occasions where other powerful technologies have been stated to be impossible so here’s Ernest Rutherford on September 11 1933 he gave a speech in Leicester addressing the British Association for the Advancement of science and he said that essentially there’s no chance that we’ll ever be able to extract energy from atoms they knew the energy was in there they could calculate how much but they said there’s no possibility we’ll ever be able to get it out and even Einstein was extremely doubtful that we would ever be able to get anything out of out of the atoms and then the next morning lady lard read about this speech in the time and went for a walk and invented the neutron induced nuclear chain reaction so so there’s there’s there’s only been like a few of these giant tech technological step changes in our history and and this one took 16 hours so so to say that you know maybe this is the fifth or sixth one that we’re talking about to say that it’s never going to happen and to be completely confident that we therefore need to take no precautionary measures whatsoever seems a little rash okay so there’s lots of other arguments
Reasons not to pay attention
I’m not going to go through them all I’ll just just page through them I did want to mention the last one the last one which is it’s very pernicious which is um so it doesn’t show up very well but don’t mention the risks it might be bad for funding so I’ve seen this not quite frequently in recent years and if you just look at what half of a nuclear power I go back to the 50s and 60s where they were trying to gain acceptance for nuclear power there was every attempt to play down all possible risks say it’s completely safe it’ll be you know too cheap to meter the electricity will be free there’s no pollution there’s no possibility of there ever being an accident and what that leads to is a lack of attention to the risks which then leaves the Chernobyl which then destroys the entire nuclear industry so history shows it’s exactly the other way around that if you suppress the risks you will destroy technological progress because then the risk will come to pass okay so I hope that you are now convinced that there is a problem and so what are we going to do about it because that’s the other thing right okay yes I agree with you this is the George Bush response okay yeah global warming is going to happen but it’s too late to do anything about it now what are we going to do about this so the work I’m going to talk about now is happening under a new center that max mentioned the center for human compatible AI at Berkeley which is funded by the open philanthropy project and what we’re basically trying to do is to change the way that we think about AI away from this notion of pure intelligence that the pure optimizer that can take any objective you like and just optimize it and naturally look at a more comprehensive kind of system which is guaranteed to be beneficial to the user in some sense there’s a lot of other work that I don’t have time to talk about many of these new centers and also those professional societies have started to become very interested in these problems as well as the funding agencies and industry so the work at the center is based on three simple ideas
Three simple ideas
the first of all that the robots only objective should be to maximize the realization of human values and the second point is the robot doesn’t know what those are but nonetheless its objective is to do this so these two points together it turns out actually makes a significant difference to how we design AI systems and the properties that they have so obviously if the robot has no idea about what human values are and never discovers what they are that’s not going to be very useful to us so it has to have some means of learning and the best source of information about human values is human behavior that the standard idea a longstanding idea in economics for example that our actions reveal our preferences and this allows for a process that results in value alignment and joshua mentioned on one of his slide
Value alignment
a fairly old idea 20 years old now inverse reinforcement learning which is the opposite of reinforcement learning or the dual so in reinforcement learning we provide a reward signal on the system has to figure out how to behave in inverse reinforcement learning we provide the behavior in other words the Machine sees our behavior and has to figure out what is the reward function that’s being optimized by this behavior so in economics this is known as structural estimation of MVPs which is a somewhat of a mouthful in control theory inverse optimal control so it’s a fairly an idea that sprung up independently in several different disciplines and there’s now a fairly well advanced theory lots and lots of papers demonstrations that this technique can be successful in learning lots of different kinds of behaviors so it isn’t quite what we want for one thing we don’t want the robot to to learn our value function and adopt it so if it sees me drinking coffee I don’t want the robot to want coffee because that’s not the that’s not the right thing that we want to happen we want the robot to know that I want coffee and to have the objective of getting me coffee whatever it might be so a slight generalization
Cooperative inverse reinforcement learning
is cooperative inverse reinforcement learning which is which is a two-player game in generally there will be many humans and many robots but to start with one human and one robot and the human in some sense knows their own value function but only in that they can act approximately according to it doesn’t mean they can explicate it and write it down and give it to the robot but they have there’s some connection between their value function and their actions the robot doesn’t know what it is but its objective is as I said before to maximize the human value function so when you write down simple instances of this game then you can solve it mathematically you can look at how the system’s behave as they play this game and as you would hope some nice things happen so the robot now has an incentive to ask questions first so it doesn’t just do whatever it thinks is best it can ask you know is this a good idea I can ask you know which of these two things might I do and the human now has an incentive to teach the robot because by teaching the robot the robot will become more useful and so the behavior of both parties is considerably changed by being in this game so I want to look at one particular instance of this game which we call the off switch problem and the opposite problem arises because based on the argument of instrumental goals the idea that you can’t fetch the coffee if you’re dead any attempt to switch off a robot you know is going to result in countermeasures by the robot and this seems like a problem that’s almost unavoidable right we said you know for pretty much any objectives very hard to think of an objective that you can carry out better after you’re dead than before it and so this is a fundamental problem and Turing’s assumption that we could just switch off the super intelligent machine is kind of like saying well you know if you’re worried about losing the game of chess you can just beat the blue just play better move right it’s not as easy as that but there is an answer which is that the robot should not be given a specific objective we want the robot to be humble in the sense the robot should know that it does not know the true objective and then it’s single-minded pursuit of the objective and it’s self defense against any interference will actually evaporate so if if the human is going to switch off the robot why would it do that the reason is that the robot is doing something that the human doesn’t like the robot of course thinks that what it’s doing is what the human likes but it acknowledges that there’s a possibility that it’s wrong and so if the human is going to switch the robot off the robot learns in some sense for being switched off that what it was doing was undesirable and therefore being switched off is better for its objective which is to optimize the human value function and so now the robot actually has a positive incentive to allow itself to be switched off it does not have a positive incentive to switch itself off so I won’t commit suicide but it will allow the human to switch it off and this is a very straightforward analog of the theorem of the non negative expected value of information but in some sense the humans action is switching off is a form of information and the robot welcomes that if it happens so this leads actually to sort of a rethinking a little bit of of how we go about doing AI that that uncertainty and objectives turns out to be quite important and it’s been ignored
Uncertainty in objectives
even though uncertainty and all kinds of other parts of AI has been studied intensively since the early 80s uncertainty in the objectives has been almost completely ignored one reason is that in the standard formulation of decision problems Markov decision processes and so on uncertainty in objectives is actually provably irrelevant because you’re trying to optimize an expected reward and if there’s uncertainty over the reward then you can simply integrate over the uncertainty and your behavior will be exactly the same as if you knew the expected value of that reward but that theorem only holds if the environment contains no information about the reward so as soon as the environment can provide more information then that theorem is invalid and clearly if what you care about is the human value function and a human is in your environment and the human can act then those actions provide information about the reward function similarly the human one particular kind of action is the provision of reward signal so reinforcement learning can occur by humans providing a reward signal now let’s go and look at a little bit of a little bit of history a reward signal and so here here are some well-known
Reward signals
experiments on what Kauai aheading so a rat will actually sort of circumvent its normal behavior and will actually starve itself to death if you give the ability to the rat to basically provide reward signals directly either by chemicals or by electrical stimulation even though it’s starving to death it will do that instead of eating so a human actually will behave the same way these are some very interesting experiments in 1950s so in any real situation unlike the mathematical model of reinforcement learning where the reward signal is provided exhaustion ously sort of as it were by God in the real world someone has to provide the reward signal you if you’re providing the reward signal are part of the environment and the reinforcement learning agent will hijack the reward generating mechanism and if that’s you then it will hijack you and force you to provide a maximal reward but this actually just results from a mistake the masam and it’s interesting that by looking at it from this different perspective from the perspective of cooperative inverse reinforcement learning we realize that the standard formulation of reinforcement learning is just wrong the signal given to the agent is not a reward it is information about the reward and you just change the mathematical formulation to have that definition instead and then the hijacking becomes completely pointless because if you hijack something that’s providing information all you do is get less information you don’t get more reward you get less information and so we can avoid wire heading by reformulating RL to have a information base rather than reward based signals so that leads to a general approach that we’re taking within the sensor that when we define a formal problem what we are
Provably beneficial AI
going to do is build agents that are designed mathematically to solve that problem and then we want to understand do those agents behave in ways that make us happy so we are not trying to solve the problem that someone else is building a an AGI from generally intelligent agent and then we are going to somehow defend against it like that’s not the right way to think the right way you think is to find a formal problem build agents that fold it and they can solve it arbitrarily well they can be arbitrarily brilliant but they are fall with all that problem F and then show that the human will benefit from having such a such a machine okay so this is a difficult problem there is a lot of
Reasons for optimism
information about human behavior in our history everything we write down is a record of human behavior so there’s a massive amount of data that we’re not really using that can be used to learn about what the human value system is so that’s good there’s also a strong economic incentive so Google found out for example if you if you write down your value function and say that the costs of misclassification of one type of object as another are all equal for every type of object and everything you could miss classify it as that value function is wrong and then you lose a lot of a lot of reputation as a result you can also imagine that you know a few years down the road mistakes in understanding human value functions will cause a very significant backlash against in the industry that produced that mistake so this is very strong incentive to get this value system right even before we reach the stage of having superhuman AI there are some reasons for
Reasons for working hard
pessimism which I always translate into reasons for working hard and that is that humans are very complicated they are not optimizers they are very complicated systems that there are lots of them and they’re all different and some of them you don’t really want to learn from and so on these are problems where we need social science to help us and it makes things much more difficult but also much more interesting so I recommend that we work on practical
Practical projects
projects that we don’t simply speculate about what a GIS might be like and and and write sort of interesting but ultimately our implementable ideas about how we might control them that we actually look at practical projects on real systems so I think with an authentic we’ll probably be looking at intelligent personal assistant other people might look at things like smart homes where clearly there are things that could go wrong and there are there are incentives to get this right early on it would be nice to have simulation environments where in fact real simulated disasters could happen so we can get more of a sense this will be a sort of a generator of ideas about what can go wrong and then how we might try to address it so I think yan is getting impatient so we’re really aiming at a change in the way that AI defines itself so we shouldn’t be talking about safe AI or beneficial
Questions
AI any more than a civil engineer talks about building bridges that don’t fall down it’s just part of the definition of a bridge that it doesn’t fall down it should be part of the definition of AI that is beneficial that it’s safe and this is not a separate AI safety community that’s nagging the real AI community this should be what they I community does intrinsically and in a state of a business we want social scientists to be involved we would like to understand a lot more about the actual human value system because it really matters we’re not building AI to benefit bacteria we’re doing AI to benefit us and hopefully it’ll make us better people that will learn a lot more about what our value systems are or could be and by making that a bit more explicit it’ll be easier for us to be good so to speak so Weiner Wiener, contd. going back to his paper from 1960 and going back to the slightly more gloomy color of the earlier part of the talk he pointed out that this is incredibly difficult to do but we don’t have a choice right we can’t just say oh this is too far off in the future too difficult to make any predictions we have to you know just continue as if nothing was going to happen the problem is bigger and more difficult but that still doesn’t mean we should ignore it thank you Thanks I was very sure you liked your formulation of the problem as a two-player cooperative game and addressing the question about switching off AI possibly I don’t think I understood it entirely though how is that particular choice by humans different than other choices if you say I want to move the bishop here I want to push the throttle forward I want to drive off the bridge I want to take the red pill how does the AI know oh this is one where I let the human overrule and this other one maybe I shouldn’t let the human decide is there a distinction between that choice and other choices if you’re uncertain yeah there is I mean I think and it also depends on your on your model of the human so if you if you have a self-driving car it has an off switch but if a self-driving car is transporting a you know a two-year-old you may want you may want to disable the off switch it’s like that’s the right thing to do so you can calculate the expected value to the human of of having an off switch that type six right now sometimes yeah sometimes the officer should be disabled so so the whole the whole evaluation is to look at the value of the human plus the robot system is that is the human better off with the robot or better off not with the robot and we only want robots where the human is provably better off and sometimes that means the robot shouldn’t allow itself to be switched off so we know a robot anesthesiologist that’s keeping you alive while you’re unconscious right is not relying on any of the decisions that you’re making right and you want to you want to trust it completely while you’re unconscious so it depends on the circumstance but the off switch problem right that curing originally proposed is a intelligent human who thinks they can switch off the machine but turns out they can’t one more question Andy Andy McAfee MIT this is an on/off switch question you brought up the great example of nuclear power as a case where maybe under discussion of risks got the field into trouble the next example in your slide was GMOs which seems like a really interesting case in click the opposite direction where the scientific consensus about safety there is at least as strong as the consensus for chemical human human caused global warming and yet a lot of that that technology is not diffusing and a lot of people are made much worse off because of over emphasis on the risk could you comment on why you added GMOs to that slide and what you conclude from it yeah I I I thought I thought through this this case and partly so in fact the the Prime Minister’s office in the UK asked me to go talk to them because they were worried that AI might be subject to the same negative outcome that happened with with GMOs in Europe I don’t think there’s any real danger of that I mean the the level of investment in the the acceleration of investment in AI is is enormous and it’s also it’s a different type of thing it’s very hard to ban AI which is really people writing formulas on whiteboards it’s not it’s not a particular organism or or a particular chemical that you could put on the field or not put on the field but I think what happened with GMOs is that the industry went into a defensive mode where they would deny any and all possible risks and so it ended up not being of it not being seen as an honest discussion that was going on it was very much the industry versus the people who had doubts or questions were trashed people were mr. industry with funding other people outside a supposedly outside to write negative articles smearing the people who are doing the research but might raise questions about GMOs so it was a they adopted the classic technique that for example the tobacco industry adopted and I think that resulted in skepticism but they’re right but it was the techniques that they adopted in the discussion right to deny risk to say that all these questions are answered trust us we know well in fact there were things they didn’t know and it’s turned out that the some of the negative sex people predicted haven’t come to pass but it wasn’t that they had already done all the science they just simply denied that anything could possibly go wrong so I think that it was more of a political failure and the political failure was not that they were they were right and everyone else was stupid it’s that they adopted this approach that everyone else was stupid and that they were right and that didn’t work