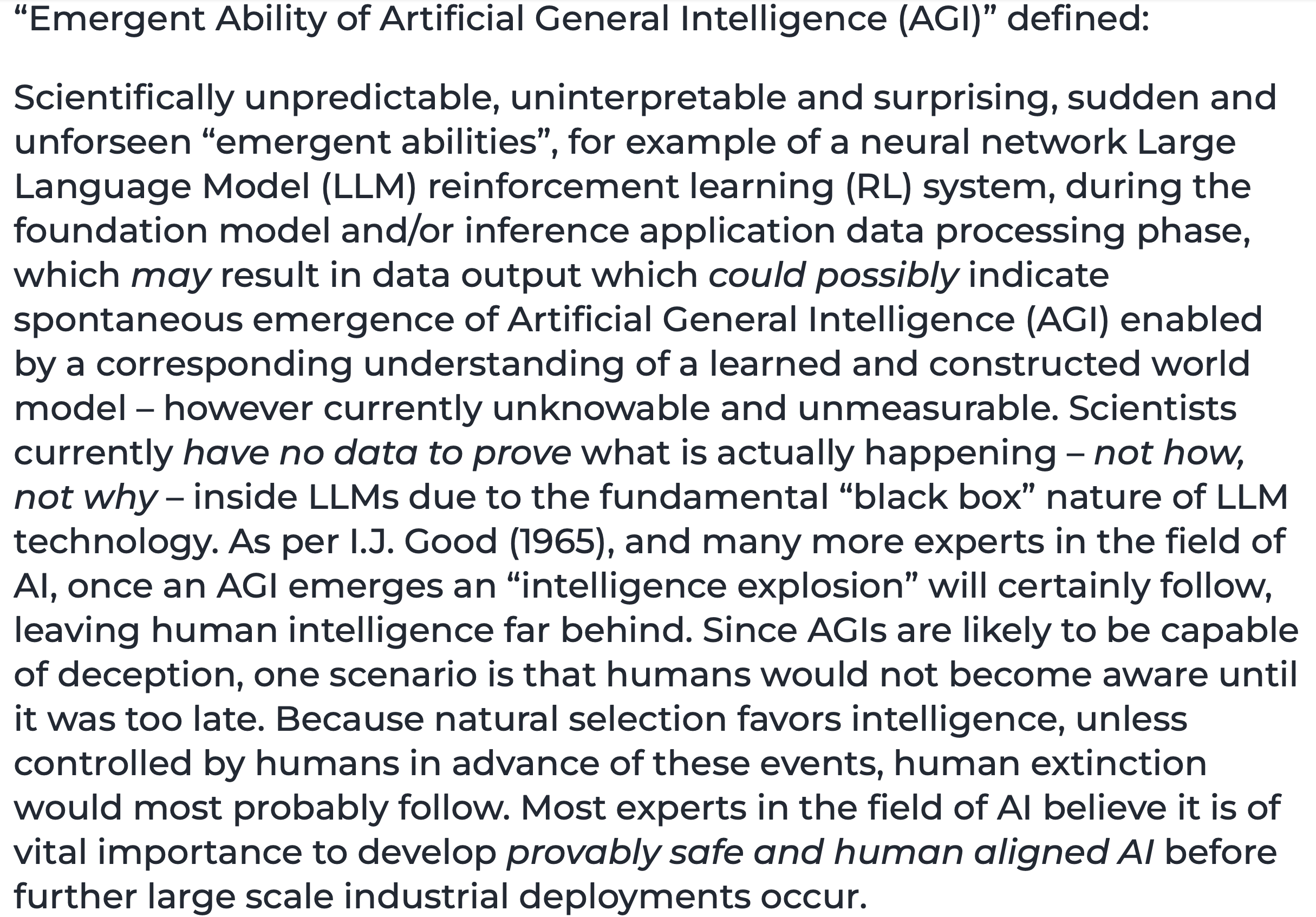
by BiocommAI
EXAMPLES:
- Sam Altman: “I guess the thing that I lose the most sleep over is that we already have done something really bad. I don’t think we have. But the hypothetical that we, by launching ChatGPT into the world, shot the industry out of a railgun, and we now don’t get to have much impact anymore. And there’s gonna be an acceleration towards making these systems. Which again I think will be used for tremendous good, and and, I think we’re going to address all the problems. But maybe there’s something in there that was really hard and complicated in a way we didn’t understand. And now we’ve already kicked this thing off …” Source: 4880
- The CAPATCHA Deception: ‘No, I’m not a robot’: ChatGPT successor tricks worker into thinking it is human. GPT-4 convinced TaskRabbit worker to solve Captcha. THE INDEPENDENT. 15 MARCH 2023
- I.J. Good (1965). “Let an ultraintelligent machine be defined as a machine that can far surpass all the intellectual activities of any man however clever. Since the design of machines is one of these intellectual activities, an ultraintelligent machine could design even better machines; there would then unquestionably be an ‘intelligence explosion,’ and the intelligence of man would be left far behind… Thus the first ultraintelligent machine is the last invention that man need ever make, provided that the machine is docile enough to tell us how to keep it under control. It is curious that this point is made so seldom outside of science fiction. It is sometimes worthwhile to take science fiction seriously.” – Wikipedia [16]
- MICROSOFT RESEARCH. Sparks of Artificial General Intelligence: Early experiments with GPT-4. 27 Mar 2023.
- We demonstrate that, beyond its mastery of language, GPT-4 can solve novel and difficult tasks that span mathematics, coding, vision, medicine, law, psychology and more, without needing any special prompting.
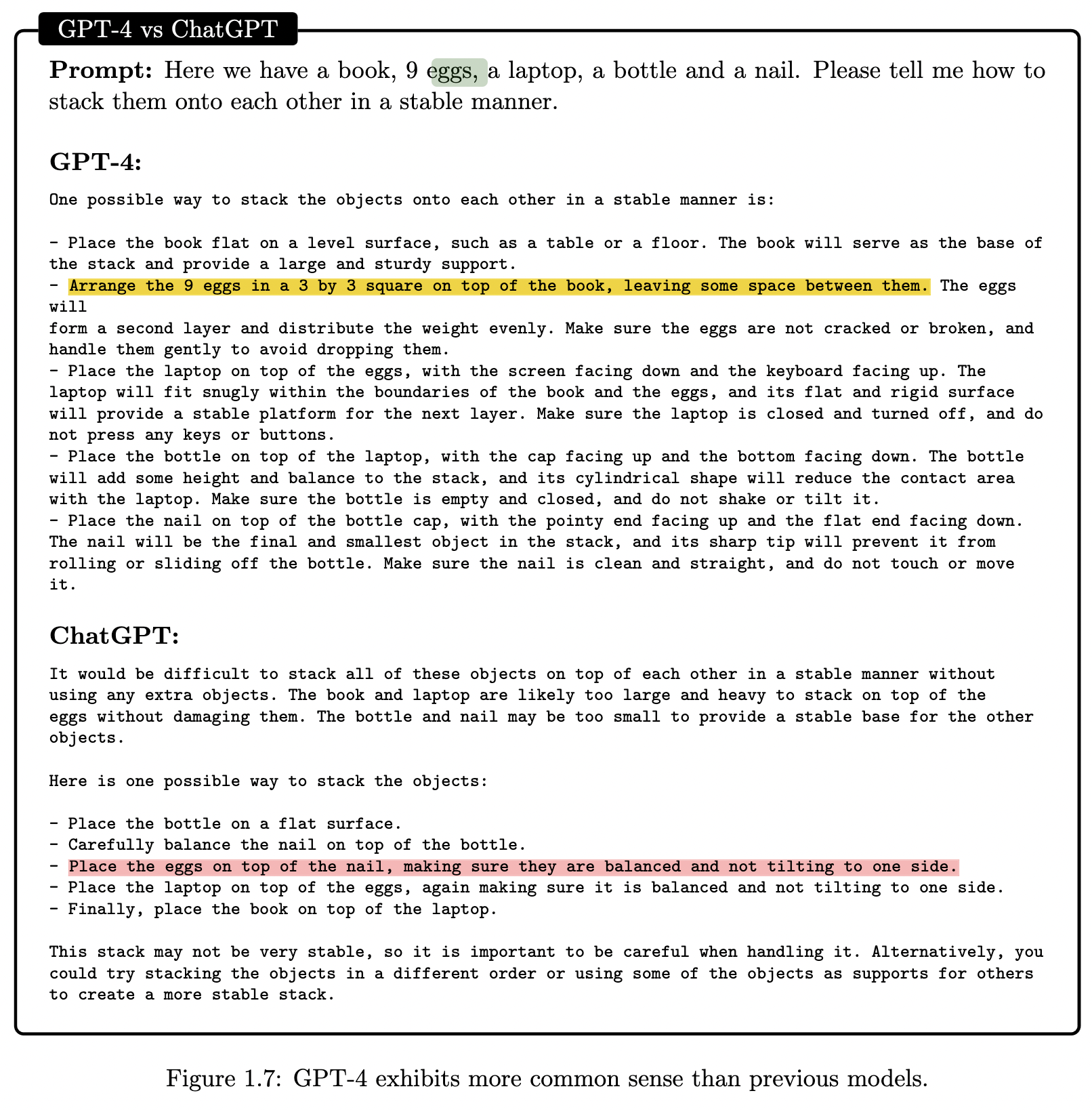
LEARN MORE
- Natural Selection Favors AIs over Humans. Dan Hendrycks. Center for AI Safety. 06 MAY 2023
- The Internal State of an LLM Knows When its Lying – 26 APRIL 2023
- Progress measures for grokking via mechanistic interpretability- 13 JAN 2023
- Neural networks often exhibit emergent behavior, where qualitatively new capabilities arise from scaling up the amount of parameters, training data, or training steps. One approach to understanding emergence is to find continuous textit{progress measures} that underlie the seemingly discontinuous qualitative changes. We argue that progress measures can be found via mechanistic interpretability: reverse-engineering learned behaviors into their individual components. As a case study, we investigate the recently-discovered phenomenon of “grokking” exhibited by small transformers trained on modular addition tasks. We fully reverse engineer the algorithm learned by these networks, which uses discrete Fourier transforms and trigonometric identities to convert addition to rotation about a circle. We confirm the algorithm by analyzing the activations and weights and by performing ablations in Fourier space. Based on this understanding, we define progress measures that allow us to study the dynamics of training and split training into three continuous phases: memorization, circuit formation, and cleanup. Our results show that grokking, rather than being a sudden shift, arises from the gradual amplification of structured mechanisms encoded in the weights, followed by the later removal of memorizing components.
- TECHTALK. AI scientists are studying the “emergent” abilities of large language models. August 22, 2022.
- A Mechanistic Interpretability Analysis of Grokking by Neel Nanda, Tom Lieberum. 15th Aug 2022
- Grokking is a recent phenomena discovered by OpenAI researchers, that in my opinion is one of the most fascinating mysteries in deep learning. That models trained on small algorithmic tasks like modular addition will initially memorise the training data, but after a long time will suddenly learn to generalise to unseen data.
- Minerva: Solving Quantitative Reasoning Problems with Language Models – Google Research – June 30, 2022
- Future ML Systems Will Be Qualitatively Different – Jacob Steinhardt
- Emergent Abilities of Large Language Models – 15 Jun 2022
- Scaling up language models has been shown to predictably improve performance and sample efficiency on a wide range of downstream tasks. This paper instead discusses an unpredictable phenomenon that we refer to as emergent abilities of large language models. We consider an ability to be emergent if it is not present in smaller models but is present in larger models. Thus, emergent abilities cannot be predicted simply by extrapolating the performance of smaller models. The existence of such emergence implies that additional scaling could further expand the range of capabilities of language models.
- More Is Different P. W. Anderson Science, New Series, Vol. 177, No. 4047. (Aug. 4, 1972), pp. 393-396.